1Departamento de Análisis Matemático, Universidad Complutense de Madrid, 28040 Madrid, Spain
2Instituto de Ciencias Matemáticas (CSIC-UAM-UC3M-UCM), 28049 Madrid, Spain
Find this paper interesting or want to discuss? Scite or leave a comment on SciRate.
Abstract
Tensor networks are factorizations of high-dimensional tensors into networks of smaller tensors. They have applications in physics and mathematics, and recently have been proposed as promising machine learning architectures. To ease the integration of tensor networks in machine learning pipelines, we introduce TensorKrowch, an open source Python library built on top of PyTorch. Providing a user-friendly interface, TensorKrowch allows users to construct any tensor network, train it, and integrate it as a layer in more intricate deep learning models. In this paper, we describe the main functionality and basic usage of TensorKrowch, and provide technical details on its building blocks and the optimizations performed to achieve efficient operation.
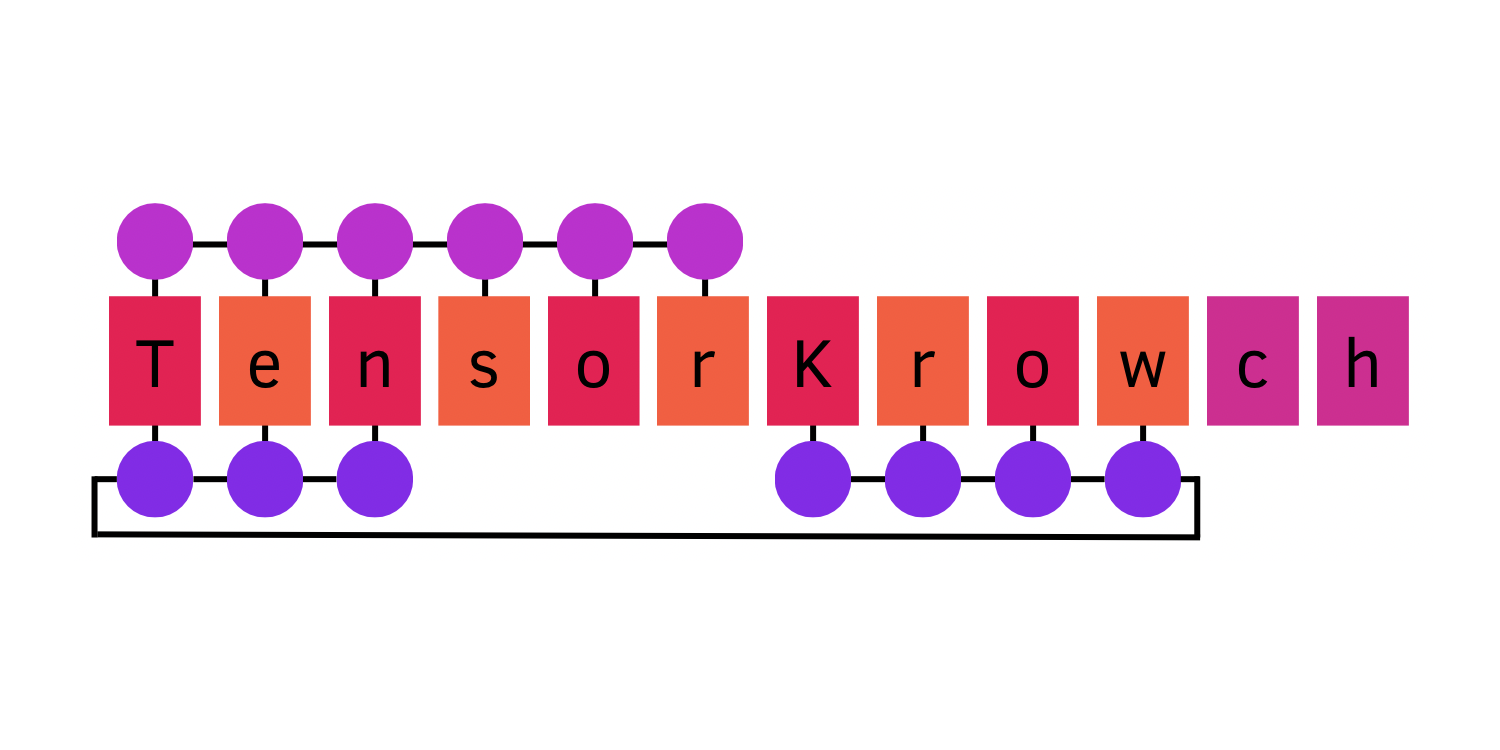
Please also see Tensor Networks with PyTorch at github.
Popular summary
This work introduces TensorKrowch, a software library for building and training machine learning models based on tensor networks. It is built on top of the popular library PyTorch, which ensures seamless integration with standard machine learning pipelines.
► BibTeX data
► References
[1] M. Fannes, B. Nachtergaele, and R. F. Werner, Commun. Math. Phys. 144, 443 (1992).
https://doi.org/10.1007/BF02099178
[2] S. R. White, Phys. Rev. Lett. 69, 2863 (1992).
https://doi.org/10.1103/PhysRevLett.69.2863
[3] G. Vidal, Phys. Rev. Lett. 91, 147902 (2003), arXiv:quant-ph/0301063.
https://doi.org/10.1103/PhysRevLett.91.147902
arXiv:quant-ph/0301063
[4] D. Pérez-García, F. Verstraete, M. M. Wolf, and J. I. Cirac, Quantum Inf. Comput. 7, 401 (2007), arXiv:quant-ph/0608197.
https://doi.org/10.26421/QIC7.5-6-1
arXiv:quant-ph/0608197
[5] G. Vidal, Phys. Rev. Lett. 99, 220405 (2007), arXiv:cond-mat/0512165.
https://doi.org/10.1103/PhysRevLett.99.220405
arXiv:cond-mat/0512165
[6] G. Vidal, Phys. Rev. Lett. 101, 110501 (2008), arXiv:quant-ph/0610099.
https://doi.org/10.1103/PhysRevLett.101.110501
arXiv:quant-ph/0610099
[7] G. Evenbly and G. Vidal, Phys. Rev. B 79, 144108 (2009), arXiv:0707.1454.
https://doi.org/10.1103/PhysRevB.79.144108
arXiv:0707.1454
[8] Y.-Y. Shi, L.-M. Duan, and G. Vidal, Phys. Rev. A 74, 022320 (2006), arXiv:quant-ph/0511070.
https://doi.org/10.1103/PhysRevA.74.022320
arXiv:quant-ph/0511070
[9] L. Tagliacozzo, G. Evenbly, and G. Vidal, Phys. Rev. B 80, 235127 (2009), arXiv:0903.5017.
https://doi.org/10.1103/PhysRevB.80.235127
arXiv:0903.5017
[10] V. Murg, F. Verstraete, Ö. Legeza, and R. M. Noack, Phys. Rev. B 82, 205105 (2010), arXiv:1006.3095.
https://doi.org/10.1103/PhysRevB.82.205105
arXiv:1006.3095
[11] K. Hémery, F. Pollmann, and D. J. Luitz, Phys. Rev. B 100, 104303 (2019), arXiv:1901.05793.
https://doi.org/10.1103/PhysRevB.100.104303
arXiv:1901.05793
[12] S.-H. Lin, M. P. Zaletel, and F. Pollmann, Phys. Rev. B 106, 245102 (2022), arXiv:1908.07545.
https://doi.org/10.1103/PhysRevB.106.245102
arXiv:1908.07545
[13] T. Soejima, K. Siva, N. Bultinck, S. Chatterjee, F. Pollmann, and M. P. Zaletel, Phys. Rev. B 101, 085117 (2020), arXiv:1908.07545.
https://doi.org/10.1103/PhysRevB.101.085117
arXiv:1908.07545
[14] F. Pan, K. Chen, and P. Zhang, Phys. Rev. Lett. 129, 090502 (2022), arXiv:2111.03011.
https://doi.org/10.1103/PhysRevLett.129.090502
arXiv:2111.03011
[15] C. Oh, M. Liu, Y. Alexeev, B. Fefferman, and L. Jiang, Tensor network algorithm for simulating experimental Gaussian boson sampling (2023), arXiv:2306.03709.
arXiv:2306.03709
[16] S. Sánchez-Ramírez, J. Conejero, F. Lordan, A. Queralt, T. Cortes, R. M. Badia, and A. García-Saez, in 2021 IEEE/ACM Second International Workshop on Quantum Computing Software (QCS) (2021) pp. 1–8, arXiv:2201.06620.
https://doi.org/10.1109/QCS54837.2021.00004
arXiv:2201.06620
[17] U. Schollwöck, Rev. Mod. Phys. 77, 259 (2005), arXiv:cond-mat/0409292.
https://doi.org/10.1103/RevModPhys.77.259
arXiv:cond-mat/0409292
[18] I. V. Oseledets, SIAM J. Sci. Comput. 33, 2295 (2011a).
https://doi.org/10.1137/090752286
[19] I. V. Oseledets, Comput. Method Appl. Math. 11, 382 (2011b).
https://doi.org/10.2478/cmam-2011-0021
[20] S. V. Dolgov and D. V. Savostyanov, Comput. Phys. Commun. 246, 106869 (2020), arXiv:1903.11554.
https://doi.org/10.1016/j.cpc.2019.106869
arXiv:1903.11554
[21] N. D. Sidiropoulos, L. De Lathauwer, X. Fu, K. Huang, E. E. Papalexakis, and C. Faloutsos, IEEE Trans. Signal Process. 65, 3551 (2017), arXiv:1607.01668.
https://doi.org/10.1109/TSP.2017.2690524
arXiv:1607.01668
[22] S. V. Dolgov and D. V. Savostyanov, Tensor product approach to modelling epidemics on networks (2022), arXiv:2209.03756.
https://doi.org/10.1016/j.amc.2023.128290
arXiv:2209.03756
[23] E. Stoudenmire and D. J. Schwab, in Advances in Neural Information Processing Systems, Vol. 29, edited by D. Lee, M. Sugiyama, U. Luxburg, I. Guyon, and R. Garnett (Curran Associates, Inc., 2016) pp. 4799–4807, arXiv:1605.05775.
arXiv:1605.05775
https://proceedings.neurips.cc/paper/2016/hash/5314b9674c86e3f9d1ba25ef9bb32895-Abstract.html
[24] A. Novikov, M. Trofimov, and I. V. Oseledets, Bull. Pol. Acad. Sci. Tech. Sci. 66, 789 (2018), arXiv:1605.03795.
https://doi.org/10.24425/bpas.2018.125926
arXiv:1605.03795
[25] J. I. Cirac, D. Pérez-García, N. Schuch, and F. Verstraete, Rev. Mod. Phys. 93, 045003 (2021), arXiv:2011.12127.
https://doi.org/10.1103/RevModPhys.93.045003
arXiv:2011.12127
[26] I. Glasser, N. Pancotti, and J. I. Cirac, IEEE Access 8, 68169 (2020), arXiv:1806.05964.
https://doi.org/10.1109/ACCESS.2020.2986279
arXiv:1806.05964
[27] J. Miller, G. Rabusseau, and J. Terilla, in Proceedings of The 24th International Conference on Artificial Intelligence and Statistics, Proceedings of Machine Learning Research, Vol. 130, edited by A. Banerjee and K. Fukumizu (PMLR, 2021) pp. 3079–3087, arXiv:2003.01039.
arXiv:2003.01039
https://proceedings.mlr.press/v130/miller21a.html
[28] J. Lopez-Piqueres, J. Chen, and A. Perdomo-Ortiz, Mach. Learn.: Sci. Technol. 4, 035009 (2023), arXiv:2211.09121.
https://doi.org/10.1088/2632-2153/ace0f5
arXiv:2211.09121
[29] D. Liu, S.-J. Ran, P. Wittek, C. Peng, R. Blázquez García, G. Su, and M. Lewenstein, New J. Phys. 21, 073059 (2019), arXiv:1710.04833.
https://doi.org/10.1088/1367-2630/ab31ef
arXiv:1710.04833
[30] S. Cheng, L. Wang, T. Xiang, and P. Zhang, Phys. Rev. B 99, 155131 (2019), arXiv:1901.02217.
https://doi.org/10.1103/PhysRevB.99.155131
arXiv:1901.02217
[31] T. Vieijra, L. Vanderstraeten, and F. Verstraete, Generative modeling with projected entangled-pair states (2022), arXiv:2202.08177.
arXiv:2202.08177
[32] F. Verstraete, M. M. Wolf, D. Pérez-García, and J. I. Cirac, Phys. Rev. Lett. 96, 220601 (2006), arXiv:quant-ph/0601075.
https://doi.org/10.1103/PhysRevLett.96.220601
arXiv:quant-ph/0601075
[33] J. Wang, C. Roberts, G. Vidal, and S. Leichenauer, Anomaly detection with tensor networks (2020), arXiv:2006.02516.
arXiv:2006.02516
[34] A. Novikov, D. Podoprikhin, A. Osokin, and D. P. Vetrov, in Advances in Neural Information Processing Systems, Vol. 28, edited by C. Cortes, N. Lawrence, D. Lee, M. Sugiyama, and R. Garnett (Curran Associates, Inc., 2015) arXiv:1509.06569.
arXiv:1509.06569
https://proceedings.neurips.cc/paper_files/paper/2015/hash/6855456e2fe46a9d49d3d3af4f57443d-Abstract.html
[35] V. Lebedev, Y. Ganin, M. Rakhuba, I. Oseledets, and V. Lempitsky, Speeding-up convolutional neural networks using fine-tuned CP-decomposition (2014), arXiv:1412.6553.
arXiv:1412.6553
[36] X. Ma, P. Zhang, S. Zhang, N. Duan, Y. Hou, M. Zhou, and D. Song, in Advances in Neural Information Processing Systems, Vol. 32, edited by H. Wallach, H. Larochelle, A. Beygelzimer, F. d’Alché-Buc, E. Fox, and R. Garnett (Curran Associates, Inc., 2019) arXiv:1906.09777.
arXiv:1906.09777
https://proceedings.neurips.cc/paper_files/paper/2019/hash/dc960c46c38bd16e953d97cdeefdbc68-Abstract.html
[37] K. Zhang, C. Hawkins, X. Zhang, C. Hao, and Z. Zhang, in Proceedings of the ICLR 2021 Workshop of Hardware Aware Efficient Training (2021) arXiv:2104.03420.
arXiv:2104.03420
https://haet2021.github.io/papers
[38] J. Tangpanitanon, C. Mangkang, P. Bhadola, Y. Minato, D. G. Angelakis, and T. Chotibut, New J. Phys. 24, 053032 (2022), arXiv:2112.08628.
https://doi.org/10.1088/1367-2630/ac6232
arXiv:2112.08628
[39] B. Aizpurua, S. Palmer, and R. Orús, Tensor networks for explainable machine learning in cybersecurity (2024), arXiv:2401.00867.
arXiv:2401.00867
[40] A. Pozas-Kerstjens, S. Hernández-Santana, J. R. Pareja Monturiol, M. Castrillón López, G. Scarpa, C. E. González-Guillén, and D. Pérez-García, Physics solutions for machine learning privacy leaks (2022), arXiv:2202.12319.
arXiv:2202.12319
[41] H. Xiao, K. Rasul, and R. Vollgraf, Fashion-MNIST: a novel image dataset for benchmarking machine learning algorithms (2017), arXiv:1708.07747.
arXiv:1708.07747
[42] J. Miller, TorchMPS (2019), https://github.com/jemisjoky/TorchMPS.
https://github.com/jemisjoky/TorchMPS
[43] M. Usvyatsov, R. Ballester-Ripoll, and K. Schindler, J. Mach. Learn. Res. 23, 1 (2022), arXiv:2206.11128.
arXiv:2206.11128
http://jmlr.org/papers/v23/21-1197.html
[44] J. Kossaifi, Y. Panagakis, A. Anandkumar, and M. Pantic, J. Mach. Learn. Res. 20, 1 (2019), arXiv:1610.09555.
arXiv:1610.09555
http://jmlr.org/papers/v20/18-277.html
[45] C. Roberts, A. Milsted, M. Ganahl, A. Zalcman, B. Fontaine, Y. Zou, J. Hidary, G. Vidal, and S. Leichenauer, TensorNetwork: A library for physics and machine learning (2019), arXiv:1905.01330.
arXiv:1905.01330
[46] J. Gray, J. Open Source Softw. 3, 819 (2018).
https://doi.org/10.21105/joss.00819
[47] J. R. Pareja Monturiol, D. Pérez-García, and A. Pozas-Kerstjens, TensorKrowch (2023), https://github.com/joserapa98/tensorkrowch.
https://github.com/joserapa98/tensorkrowch
[48] A. Paszke, S. Gross, F. Massa, A. Lerer, J. Bradbury, G. Chanan, T. Killeen, Z. Lin, N. Gimelshein, L. Antiga, A. Desmaison, A. Kopf, E. Yang, Z. DeVito, M. Raison, A. Tejani, S. Chilamkurthy, B. Steiner, L. Fang, J. Bai, and S. Chintala, in Advances in Neural Information Processing Systems, Vol. 32, edited by H. Wallach, H. Larochelle, A. Beygelzimer, F. d’Alché Buc, E. Fox, and R. Garnett (Curran Associates, Inc., 2019) arXiv:1912.01703.
arXiv:1912.01703
https://proceedings.neurips.cc/paper_files/paper/2019/hash/bdbca288fee7f92f2bfa9f7012727740-Abstract.html
[49] R. Orús, Ann. Phys. 349, 117 (2014), arXiv:1306.2164.
https://doi.org/10.1016/j.aop.2014.06.013
arXiv:1306.2164
[50] J. C. Bridgeman and C. T. Chubb, J. Phys. A: Math. Theor. 50, 223001 (2017), arXiv:1603.03039.
https://doi.org/10.1088/1751-8121/aa6dc3
arXiv:1603.03039
[51] T. G. Kolda and B. W. Bader, SIAM Rev. 51, 455 (2009).
https://doi.org/10.1137/07070111X
[52] B. Pirvu, V. Murg, J. I. Cirac, and F. Verstraete, New J. Phys. 12, 025012 (2010), arXiv:0804.3976.
https://doi.org/10.1088/1367-2630/12/2/025012
arXiv:0804.3976
[53] J. Martyn, G. Vidal, C. Roberts, and S. Leichenauer, Entanglement and tensor networks for supervised image classification (2020), arXiv:2007.06082.
arXiv:2007.06082
[54] J. A. Reyes and E. M. Stoudenmire, Mach. Learn.: Sci. Technol. 2, 035036 (2021), arXiv:2001.08286.
https://doi.org/10.1088/2632-2153/abffe8
arXiv:2001.08286
[55] D. G. A. Smith and J. Gray, J. Open Source Softw. 3, 753 (2018).
https://doi.org/10.21105/joss.00753
[56] V. Zauner-Stauber, L. Vanderstraeten, M. T. Fishman, F. Verstraete, and J. Haegeman, Phys. Rev. B 97, 045145 (2018), arXiv:1701.07035.
https://doi.org/10.1103/PhysRevB.97.045145
arXiv:1701.07035
[57] J. Gray and S. Kourtis, Quantum 5, 410 (2021), arXiv:2002.01935.
https://doi.org/10.22331/q-2021-03-15-410
arXiv:2002.01935
[58] A. Acuaviva, V. Makam, H. Nieuwboer, D. Pérez-García, F. Sittner, M. Walter, and F. Witteveen, in 2023 IEEE 64th Annual Symposium on Foundations of Computer Science (FOCS) (2023) pp. 328–362, arXiv:2209.14358.
https://doi.org/10.1109/FOCS57990.2023.00027
arXiv:2209.14358
[59] C. Damm, M. Holzer, and P. McKenzie, Comput. Complex. 11, 54 (2002).
https://doi.org/10.1007/s00037-000-0170-4
[60] C. Hawkins, X. Liu, and Z. Zhang, SIAM J. Math. Data Sci. 4, 46 (2022), arXiv:2010.08689.
https://doi.org/10.1137/21M1391444
arXiv:2010.08689
[61] N. Kargas and N. D. Sidiropoulos, IEEE Trans. Signal Process. 69, 1097 (2021).
https://doi.org/10.1109/TSP.2021.3055000
[62] Y. Liu and M. K. Ng, Knowl.-Based Syst. 241, 108171 (2022).
https://doi.org/10.1016/j.knosys.2022.108171
Cited by
This Paper is published in Quantum under the Creative Commons Attribution 4.0 International (CC BY 4.0) license. Copyright remains with the original copyright holders such as the authors or their institutions.
- SEO Powered Content & PR Distribution. Get Amplified Today.
- PlatoData.Network Vertical Generative Ai. Empower Yourself. Access Here.
- PlatoAiStream. Web3 Intelligence. Knowledge Amplified. Access Here.
- PlatoESG. Carbon, CleanTech, Energy, Environment, Solar, Waste Management. Access Here.
- PlatoHealth. Biotech and Clinical Trials Intelligence. Access Here.
- Source: https://quantum-journal.org/papers/q-2024-06-11-1364/
- :has
- :is
- ][p
- 06
- 1
- 10
- 100
- 11
- 12
- 13
- 130
- 14
- 15%
- 16
- 17
- 19
- 20
- 2001
- 2005
- 2006
- 2008
- 2009
- 2011
- 2014
- 2015
- 2016
- 2017
- 2018
- 2019
- 2020
- 2021
- 2022
- 2023
- 2024
- 22
- 23
- 24
- 24th
- 25
- 26%
- 27
- 28
- 29
- 30
- 31
- 32
- 33
- 35%
- 36
- 37
- 39
- 40
- 41
- 43
- 455
- 49
- 50
- 51
- 53
- 54
- 58
- 60
- 61
- 65
- 66
- 69
- 7
- 750
- 77
- 8
- 80
- 9
- 91
- 97
- a
- ABSTRACT
- access
- Achieve
- advances
- ADvantage
- advantages
- affiliations
- against
- algorithm
- algorithms
- allows
- also
- alt
- AMC
- an
- and
- ann
- annual
- anomaly detection
- any
- applications
- approach
- architectures
- ARE
- areas
- artificial
- artificial intelligence
- AS
- associates
- At
- author
- authors
- aware
- based
- basic
- been
- benchmarking
- Blocks
- boson
- Break
- Building
- built
- bull
- by
- CAN
- cases
- chen
- Cheng
- Chubb
- claims
- classification
- comment
- Commons
- complex
- computer
- computer science
- computing
- Conference
- construct
- conventional
- copyright
- Cybersecurity
- data
- David
- de
- deep
- deep learning
- describe
- details
- Detection
- discuss
- e
- ease
- efficiency
- efficient
- ensures
- entanglement
- experimental
- fact
- For
- Foundations
- fox
- fu
- functionality
- generative
- GitHub
- gray
- gross
- Hardware
- Have
- High
- holders
- HTML
- http
- HTTPS
- huang
- i
- IEEE
- image
- Image classification
- in
- Inc.
- information
- institutions
- integrate
- integration
- Intelligence
- interesting
- Interface
- International
- into
- intricate
- introduce
- Introduces
- IT
- ITS
- JavaScript
- journal
- known
- lawrence
- layer
- Leaks
- LEARN
- learning
- Leave
- Lee
- Library
- License
- lin
- machine
- machine learning
- Main
- math
- mathematics
- max-width
- method
- Miller
- modeling
- modelling
- models
- Month
- more
- network
- networks
- neural
- neural networks
- New
- novel
- now
- of
- oh
- on
- open
- open source
- operation
- optimizations
- or
- original
- Outperform
- pages
- Palmer
- Paper
- performed
- Physics
- plato
- Plato Data Intelligence
- PlatoData
- Popular
- powerful
- preservation
- privacy
- Proceedings
- process
- processing
- Product
- projected
- promising
- proposed
- provide
- providing
- published
- publisher
- Python
- pytorch
- Quantum
- quantum advantage
- quantum computing
- R
- recently
- references
- remains
- research
- s
- SCI
- Science
- seamless
- Second
- see
- siam
- Signal
- smaller
- smith
- smooth
- Software
- Solutions
- song
- Source
- specific
- standard
- States
- statistics
- Studying
- such
- Symposium
- Systems
- tech
- Technical
- that
- The
- their
- There.
- they
- this
- Title
- to
- tools
- top
- Train
- Training
- under
- URL
- Usage
- use
- user-friendly
- users
- using
- very
- volume
- W
- wang
- want
- we
- which
- white
- with
- Wolf
- Work
- workshop
- X
- xiao
- year
- zephyrnet