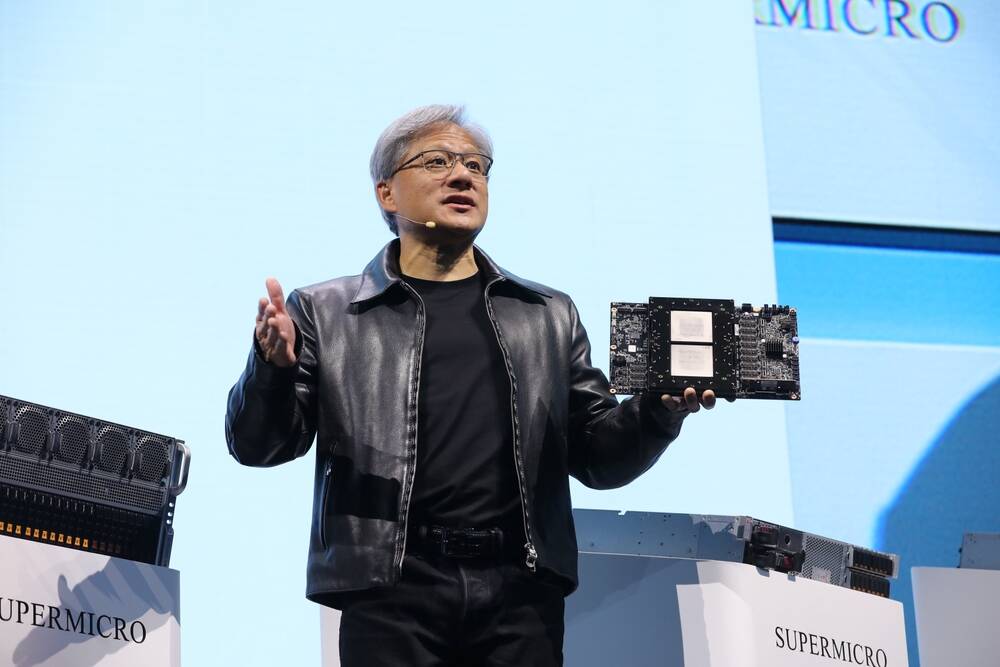
komentar Nvidia’s latest quarter marked a defining moment for AI adoption.
Demand for the tech titan’s GPUs drove its revenues to nove višine, saj so se podjetja, ponudniki oblakov in hiperskalerji vsi trudili, da bi ostali ustrezni v novem svetovnem redu umetne inteligence.
But while Nvidia’s execs expect to extract multi-billion dollar gains from this demand over the next several quarters, the question on many minds is whether Nvidia and partners can actually build enough GPUs to satisfy demand, and what happens if they can’t.
V pogovoru s finančnimi analitiki, Nvidia CFO Colette Kress zagotovljeno Wall Street that the graphics processor giant was working closely with partners to reduce cycle times and add supply capacity. When pressed for specifics, Kress repeatedly dodged the question, arguing that Nv’s gear involved so many suppliers it was difficult to say how much capacity they’d be able to bring to bear and when.
Financial Times poročilo, meanwhile, suggested Nvidia plans to, at a minimum, triple the production of its top-spec H100 accelerator in 2024 to between 1.5 and 2 million units, up from roughly half a million this year. While this is great news for Nvidia’s bottom line, if true, some companies aren’t waiting around for Nvidia to catch up, and instead are looking to alternative architectures.
Neizpolnjeno povpraševanje ustvarja priložnost
Eden najbolj prepričljivih primerov je oblak G42 Združenih arabskih emiratov, ki prisluškoval Cerebras Systems bo zgradil devet superračunalnikov z umetno inteligenco, ki bodo zmožni kombiniranih 36 eksaflopov redke zmogljivosti FP16, za zgolj 100 milijonov dolarjev na kos.
Cerebras’s pospeševalniki are wildly different from the GPUs that power Nvidia’s HGX and DGX systems. Rather than packing four or eight GPUs into a rack mount chassis, Cerebra’s accelerators are enormous dinner-plate-sized sheets of silicon packing 850,000 cores and 40GB of SRAM. The chipmaker claims just 16 of these accelerators are required to achieve 1 exaflop of sparse FP16 performance, a feat that, by our estimate, would require north of 500 Nvidia H100s.
And for others willing to venture out beyond Nvidia’s walled garden, there’s no shortage of alternatives. Last we heard, Amazon is uporabo Intel’s Gaudi AI training accelerators to supplement its own custom Trainium chips — though it isn’t clear in what volumes.
Compared to Nvidia’s A100, Intel’s Gaudi2 processors, which začela maja lani trdijo, da zagotavljajo približno dvakrat večjo zmogljivost, vsaj v modelu za klasifikacijo slik ResNet-50 in modelih obdelave naravnega jezika BERT. In za tiste na Kitajskem, Intel pred kratkim Uvedeno a cut down version of the chip for sale in the region. Intel is expected to launch an even more powerful version of the processor, predictably called Gaudi3, to compete with Nvidia’s current-gen H100 sometime next year.
Then of course, there’s AMD, which, having enjoyed a recent string of high-profile wins in the supercomputing space, has turned its attention to the AI market.
Na junijskem dogodku Datacenter and AI je AMD podrobno njegov Instinct MI300X, ki naj bi se začel pošiljati do konca leta. Pospeševalnik združuje 192 GB hitrega pomnilnika HBM3 in osem grafičnih procesorjev CDNA 3 v enem paketu.
Naša sestrska stran Naslednja platforma ocene the chip will deliver roughly 3 petaflops of FP8 performance. While 75 percent of a Nvidia’s H100 in terms of performance, the MI300X’s offers 2.4x higher memory capacity, which could allow customers to get away with using fewer GPUs to train their models.
Možnosti GPE-ja, ki ne more le zagotoviti prepričljive zmogljivosti, ampak tudi vi dejansko kupi, clearly has piqued some interest. During AMD’s Q2 earnings call this month, CEO Lisa Su hvalil that the company’s AI engagements had grown seven fold during the quarter. “In the datacenter alone, we expect the market for AI accelerators to reach over $150 billion by 2027,” she said.
Ovire za posvojitev
So if Nvidia thinks right now it’s only addressing a third of demand for its AI-focused silicon, why aren’t its rivals stepping up to fill in the gap and cash in on the hype?
The most obvious issue is that of timing. Neither AMD nor Intel will have accelerators capable of challenging Nvidia’s H100, at least in terms of performance, ready for months. However, even after that, customers will still have to contend with less mature software.
Then there’s the fact that Nvidia’s rivals will be fighting for the same supplies and manufacturing capacity that Nv wants to secure or has already secured. For example, AMD opira na TSMC tako kot Nvidia za izdelavo čipov. Čeprav je povpraševanje po polprevodnikih je v krču ker se v zadnjem času vse manj ljudi zanima za nakup osebnih računalnikov, telefonov in podobnega, obstaja veliko povpraševanje po strežniških pospeševalnikih za usposabljanje modelov in napajanje aplikacij za strojno učenje.
But back to the code: Nvidia’s close knit hardware and software ecosystem has been around for years. As a result, there’s a lot of code, including many of the most popular AI models, optimized for Nv’s industry-dominating CUDA framework.
That’s not to say rival chip houses aren’t trying to change this dynamic. Intel’s OneAPI includes tools to help users to pretvorbo code written for Nvidia’s CUDA to SYCL, which can then run on Intel’s suite of AI platforms. Similar efforts have been made to convert CUDA workloads to run on AMD’s Instinct GPU family using the HIP API.
Številni od teh istih izdelovalcev čipov prav tako prosijo za pomoč podjetja, kot je Hugging Face, ki razvija orodja za gradnjo aplikacij ML, da bi zmanjšali ovire za izvajanje priljubljenih modelov na njihovi strojni opremi. Te naložbe recently drove Hugging’s valuation to over $4 billion.
Drugi proizvajalci čipov, kot je Cerebras, so poskušali preprečiti to posebno težavo z razvojem prilagojenih modelov umetne inteligence za svojo strojno opremo, ki jih stranke lahko izkoristijo, namesto da bi morale začeti iz nič. Še marca, Cerebras razglasitve Cerebras-GPT, zbirka sedmih LLM z velikostjo od 111 milijonov do 13 milijard parametrov.
Za bolj tehnične stranke z viri, ki jih lahko namenijo razvoju, optimizaciji ali prenosu podedovane kode na novejše, manj zrele arhitekture, bo izbira alternativne platforme strojne opreme morda vredna morebitnih prihrankov pri stroških ali skrajšanih dobavnih rokov. Tako Google kot Amazon sta že šla po tej poti s svojima pospeševalnikoma TPU oziroma Trainium.
However, for those that lack these resources, embracing infrastructure without a proven software stack – no matter how performant it may be – could be seen as a liability. In that case, Nvidia will likely remain the safe bet. ®
- Distribucija vsebine in PR s pomočjo SEO. Okrepite se še danes.
- PlatoData.Network Vertical Generative Ai. Opolnomočite se. Dostopite tukaj.
- PlatoAiStream. Web3 Intelligence. Razširjeno znanje. Dostopite tukaj.
- PlatoESG. Avtomobili/EV, Ogljik, CleanTech, Energija, Okolje, sončna energija, Ravnanje z odpadki. Dostopite tukaj.
- PlatoHealth. Obveščanje o biotehnologiji in kliničnih preskušanjih. Dostopite tukaj.
- ChartPrime. Izboljšajte svojo igro trgovanja s ChartPrime. Dostopite tukaj.
- BlockOffsets. Posodobitev okoljskega offset lastništva. Dostopite tukaj.
- vir: https://go.theregister.com/feed/www.theregister.com/2023/08/29/nvidia_q2_ai_market/
- :ima
- : je
- :ne
- :kje
- $ 100 milijonov
- $GOR
- 000
- 1
- 13
- 16
- 2024
- 36
- 500
- 7
- 75
- a
- Sposobna
- plin
- pospeševalniki
- Doseči
- dejansko
- dodajte
- naslavljanje
- Sprejetje
- po
- AI
- AI modeli
- AI usposabljanje
- vsi
- omogočajo
- sam
- že
- Prav tako
- alternativa
- alternative
- Amazon
- AMD
- an
- Analitiki
- in
- API
- aplikacije
- aplikacije
- arab
- Arabski emirati
- SE
- okoli
- AS
- At
- pozornosti
- stran
- nazaj
- ovira
- BE
- Nosijo
- bilo
- Stavim
- med
- Poleg
- Billion
- tako
- Bottom
- prinašajo
- izgradnjo
- Building
- vendar
- by
- klic
- se imenuje
- CAN
- lahko
- kapaciteta
- primeru
- Denar
- wrestling
- ceo
- cfo
- izziv
- spremenite
- Kitajska
- čip
- čipi
- trdijo
- terjatve
- Razvrstitev
- jasno
- jasno
- Zapri
- tesno
- Cloud
- CO
- Koda
- zbirka
- kombinirani
- Podjetja
- podjetje
- prepričljiv
- tekmujejo
- pretvorbo
- strošek
- prihranki pri stroških
- bi
- Tečaj
- po meri
- Stranke, ki so
- Cut
- cikel
- Datacenter
- definiranje
- poda
- Povpraševanje
- razvoju
- razvija
- drugačen
- težko
- ne
- Dollar
- navzdol
- med
- dinamično
- Plače
- zaslužek klic
- ekosistem
- prizadevanja
- ostalo
- objame
- emirates
- konec
- posli
- ogromno
- dovolj
- podjetja
- oceniti
- Tudi
- Event
- vsi
- eksaflop
- Primer
- Primeri
- Execs
- pričakovati
- Pričakuje
- ekstrakt
- Obraz
- Dejstvo
- družina
- feat
- manj
- boju proti
- izpolnite
- finančna
- Financial Times
- za
- štiri
- Okvirni
- iz
- FT
- zaslužek
- vrzel
- Vrt
- Oprema
- dobili
- velikan
- več
- GPU
- Grafične kartice
- grafika
- veliko
- goji
- imel
- Pol
- se zgodi
- strojna oprema
- Imajo
- ob
- Slišal
- pomoč
- odmeven
- več
- hiše
- Kako
- Vendar
- HTTPS
- hype
- if
- slika
- Razvrstitev slik
- in
- vključuje
- Vključno
- Infrastruktura
- Namesto
- Intel
- obresti
- zainteresirani
- v
- vključeni
- isn
- vprašanje
- IT
- ITS
- jpg
- junij
- samo
- plesti
- label
- Pomanjkanje
- jezik
- Zadnja
- Zadnji
- kosilo
- vodi
- učenje
- vsaj
- Legacy
- manj
- Vzvod
- odgovornosti
- kot
- Verjeten
- vrstica
- Pogledal
- si
- Sklop
- stroj
- strojno učenje
- je
- proizvodnja
- več
- marec
- označeno
- Tržna
- Matter
- zrel
- Maj ..
- Medtem
- Spomin
- Mers
- milijonov
- misli
- minimalna
- ML
- Model
- modeli
- Trenutek
- mesec
- mesecev
- več
- Najbolj
- Najbolj popularni
- Gora
- veliko
- naravna
- Obdelava Natural Language
- Niti
- Novo
- novice
- Naslednja
- št
- sever
- zdaj
- NV
- Nvidia
- Očitna
- of
- Ponudbe
- on
- samo
- optimizirana
- optimizacijo
- or
- Da
- drugi
- naši
- ven
- več
- lastne
- paket
- Paketi
- parametri
- zlasti
- partnerji
- računalniki
- ljudje
- odstotkov
- performance
- telefoni
- kos
- načrti
- platforma
- Platforme
- platon
- Platonova podatkovna inteligenca
- PlatoData
- Popular
- potencial
- moč
- močan
- obravnavati
- Procesor
- procesorji
- proizvodnja
- možnosti
- dokazano
- ponudniki
- Q2
- četrtletje
- vprašanje
- obsegu
- precej
- dosežejo
- pripravljen
- nedavno
- Pred kratkim
- zmanjša
- Zmanjšana
- okolica
- pomembno
- ostajajo
- PONOVNO
- zahteva
- obvezna
- viri
- oziroma
- povzroči
- prihodki
- Pravica
- Tekmec
- tekmecev
- grobo
- Pot
- Run
- tek
- s
- varna
- Je dejal
- prodaja
- Enako
- Prihranki
- pravijo,
- praska
- zavarovanje
- Zavarovano
- videl
- polprevodnik
- sedem
- več
- je
- Dostava
- pomanjkanje
- strani
- pomemben
- Silicij
- Podoben
- sam
- sestra
- spletna stran
- Velikosti
- So
- Software
- nekaj
- Vesolje
- posebnosti
- hitrost
- sveženj
- Začetek
- bivanje
- Korak
- stopi
- Še vedno
- ulica
- String
- apartma
- Superračunalništvo
- dopolnjujejo
- dobavitelji
- dobavi
- sistemi
- tech
- tehnični
- Pogoji
- kot
- da
- O
- njihove
- POTEM
- Tukaj.
- te
- jih
- Misli
- tretja
- ta
- letos
- tisti,
- čeprav?
- krat
- čas
- titan
- do
- orodja
- Vlak
- usposabljanje
- Triple
- Res
- tsmc
- Obrnjen
- Dvakrat
- pod
- Velika
- Združeni arab
- Združeni Arabski Emirati
- enote
- Uporabniki
- uporabo
- Vrednotenje
- podjetje
- različica
- prostornine
- Čakam
- Wall
- Wall Street
- Stenasto
- želi
- je
- we
- Kaj
- kdaj
- ali
- ki
- medtem
- zakaj
- bo
- pripravljeni
- Zmage
- z
- brez
- deluje
- svet
- vredno
- bi
- pisni
- leto
- let
- Vi
- zefirnet