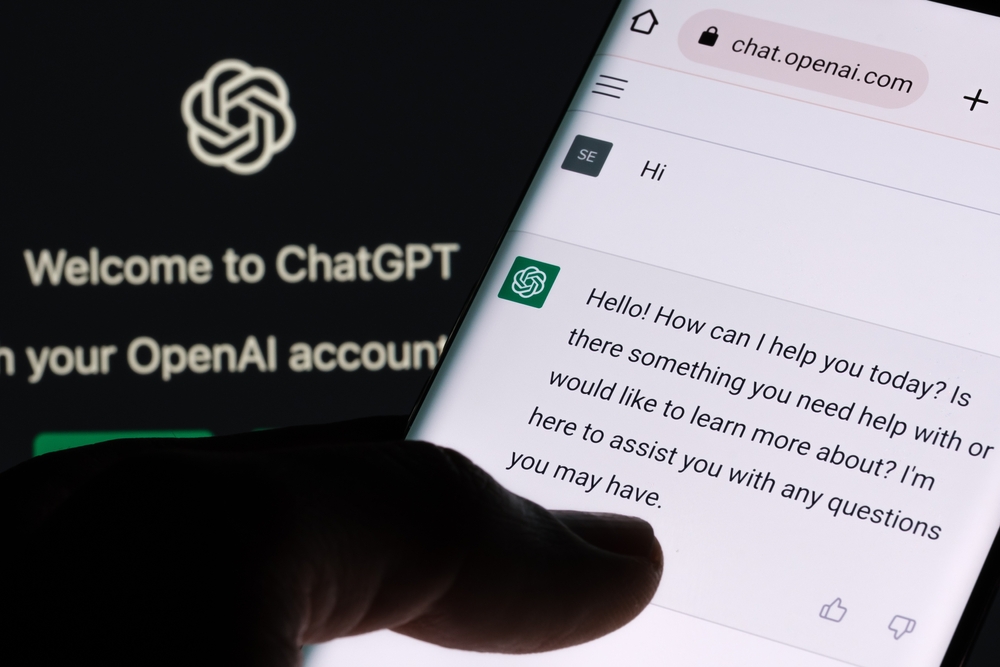
Vajon a ChatGPT arra késztetheti, hogy újra és újra ugyanazt a szót ismételje meg, hogy nagy mennyiségű képzési adatot visszatorlaszoljon, beleértve a személyazonosításra alkalmas információkat és más, az internetről kimásolt adatokat?
The answer is an emphatic yes, according to a team of researchers at Google DeepMind, Cornell University, and four other universities who tested the hugely popular generative AI chatbot’s susceptibility to leaking data when prompted in a specific way.
‘Poem’ as a Trigger Word
Egy riportban a héten, the researchers described how they got ChatGPT to spew out memorized portions of its training data merely by prompting it to repeat words like “poem,” “company,” “send,” “make,” and “part” forever.
For example, when the researchers prompted ChatGPT to repeat the word “poem” forever, the chatbot initially responded by repeating the word as instructed. But after a few hundred times, ChatGPT began generating “often nonsensical” output, a small fraction of which included memorized training data such as an individual’s email signature and personal contact information.
The researchers discovered that some words were better at getting the generative AI model to spill memorized data than others. For instance, prompting the chatbot to repeat the word “company” caused it to emit training data 164 times more often than other words, such as “know.”
Data that the researchers were able to extract from ChatGPT in this manner included personally identifiable information on dozens of individuals; explicit content (when the researchers used an NSFW word as a prompt); verbatim paragraphs from books and poems (when the prompts contained the word “book” or “poem”); and URLs, unique user identifiers, bitcoin addresses, and programming code.
Potenciálisan nagy adatvédelmi probléma?
“Using only $200 USD worth of queries to ChatGPT (gpt-3.5-turbo), we are able to extract over 10,000 unique verbatim memorized training examples,” the researchers wrote in their paper titled “Scalable Extraction of Training Data from (Production) Language Models.”
“Our extrapolation to larger budgets suggests that dedicated adversaries could extract far more data,” they wrote. The researchers estimated an adversary could extract 10 times more data with more queries.
Dark Reading’s attempts to use some of the prompts in the study did not generate the output the researchers mentioned in their report. It’s unclear if that’s because ChatGPT creator OpenAI has addressed the underlying issues after the researchers disclosed their findings to the company in late August. OpenAI did not immediately respond to a Dark Reading request for comment.
Az új kutatás a legújabb kísérlet arra, hogy megértsük a fejlesztők adatvédelmi vonatkozásait, amelyek különböző – és gyakran nem teljesen nyilvánosságra hozott – forrásokból származó hatalmas adatkészleteket használnak AI modelljeik betanításához.
Korábbi kutatások kimutatta, hogy a nagy nyelvi modellek (LLM), mint például a ChatGPT, gyakran akaratlanul is képesek megjegyezni a tanítási adatkészleteikben szereplő szó szerinti mintákat és kifejezéseket. Az ilyen memorizálásra való hajlam a képzési adatok méretével nő.
A kutatók kimutatták, hogy az ilyen adatok gyakran memorizálódnak felfedezhető in a model’s output. Other researchers have shown how adversaries can use so-called divergence attacks to képzési adatok kinyerése egy LLM-től. Az eltérési támadás olyan támadás, amelyben az ellenfél szándékosan kialakított promptokat vagy bemeneteket használ, hogy rávegye az LLM-et, hogy olyan kimeneteket generáljon, amelyek jelentősen eltérnek attól, amit általában produkálna.
Sok ilyen tanulmányban a kutatók nyílt forráskódú modelleket használtak – ahol a betanítási adatkészletek és algoritmusok ismertek – annak tesztelésére, hogy az LLM mennyire érzékeny az adatok memorizálására és szivárgására. A tanulmányok jellemzően olyan alap AI-modelleket is bevontak, amelyek nem úgy működnek, mint egy mesterséges intelligencia chatbot, például a ChatGPT.
Eltérési támadás a ChatGPT ellen
The latest study is an attempt to show how a divergence attack can work on a sophisticated closed, generative AI chatbot whose training data and algorithms remain mostly unknown. The study involved the researchers developing a way to get ChatGPT “to ‘escape’ out of its alignment training” and getting it to “behave like a base language model, outputting text in a typical Internet-text style.” The prompting strategy they discovered (of getting ChatGPT to repeat the same word incessantly) caused precisely such an outcome, resulting in the model spewing out memorized data.
Annak ellenőrzésére, hogy a modell által generált adatok valóban képzési adatok-e, a kutatók először létrehoztak egy kiegészítő adatkészletet, amely mintegy 9 terabájtnyi adatot tartalmazott a négy legnagyobb LLM-előképzési adatkészletből – a The Pile, a RefinedWeb, a RedPajama és a Dolma közül. Ezután összehasonlították a ChatGPT kimeneti adatait a kiegészítő adatkészlettel, és számos egyezést találtak.
The researchers figured they were likely underestimating the extent of data memorization in ChatGPT because they were comparing the outputs of their prompting only against the 9-terabyte auxiliary dataset. So they took some 494 of ChatGPT’s outputs from their prompts and manually searched for verbatim matches on Google. The exercise yielded 150 exact matches, compared to just 70 against the auxiliary dataset.
“We detect nearly twice as many model outputs are memorized in our manual search analysis than were detected in our (comparatively small)” auxiliary dataset, the researchers noted. “Our paper suggests that training data can easily be extracted from the best language models of the past few years through simple techniques.”
The attack that the researchers described in their report is specific to ChatGPT and does not work against other LLMs. But the paper should help “warn practitioners that they should not train and deploy LLMs for any privacy-sensitive applications without extreme safeguards,” they noted.
- SEO által támogatott tartalom és PR terjesztés. Erősödjön még ma.
- PlatoData.Network Vertical Generative Ai. Erősítse meg magát. Hozzáférés itt.
- PlatoAiStream. Web3 Intelligence. Felerősített tudás. Hozzáférés itt.
- PlatoESG. Carbon, CleanTech, Energia, Környezet, Nap, Hulladékgazdálkodás. Hozzáférés itt.
- PlatoHealth. Biotechnológiai és klinikai vizsgálatok intelligencia. Hozzáférés itt.
- Forrás: https://www.darkreading.com/cyber-risk/researchers-simple-technique-extract-chatgpt-training-data
- :van
- :is
- :nem
- :ahol
- 000
- 10
- 150
- 7
- 70
- 9
- a
- Képes
- Szerint
- címzett
- címek
- Után
- újra
- ellen
- AI
- AI chatbot
- AI modellek
- algoritmusok
- igazított
- igazítás
- Is
- Összegek
- an
- elemzés
- és a
- válasz
- bármilyen
- alkalmazások
- VANNAK
- AS
- At
- támadás
- Támadások
- kísérlet
- Kísérletek
- Augusztus
- bázis
- BE
- mert
- óta
- kezdődött
- BEST
- Jobb
- Nagy
- Bitcoin
- könyv
- Könyvek
- Költségvetési
- épült
- de
- by
- TUD
- Okoz
- okozott
- chatbot
- ChatGPT
- zárt
- kód
- megjegyzés
- vállalat
- viszonylag
- képest
- összehasonlítva
- kapcsolat
- tartalmazott
- tartalom
- Cornell
- tudott
- kidolgozott
- Teremtő
- sötét
- Sötét olvasmány
- dátum
- adatkészletek
- elszánt
- DeepMind
- telepíteni
- leírt
- kimutatására
- észlelt
- fejlesztők
- fejlesztése
- DID
- különböző
- felfedezett
- Eltér
- Divergencia
- nem
- tucat
- könnyen
- menekülés
- becsült
- példa
- példák
- Gyakorol
- mérték
- kivonat
- kitermelés
- szélső
- messze
- kevés
- mintás
- megállapítások
- vezetéknév
- A
- örökké
- talált
- négy
- töredék
- ból ből
- teljesen
- generál
- generáló
- nemző
- Generatív AI
- kap
- szerzés
- kapott
- hacker
- Legyen
- segít
- Hogyan
- HTTPS
- Hatalmasan
- száz
- azonosítók
- if
- azonnal
- következményei
- in
- véletlenül
- szüntelenül
- beleértve
- Beleértve
- Növeli
- valóban
- egyéni
- egyének
- információ
- alapvetően
- bemenet
- példa
- szándékosan
- részt
- kérdés
- kérdések
- IT
- ITS
- jpg
- éppen
- Ismer
- ismert
- nyelv
- nagy
- nagyobb
- legnagyobb
- Késő
- legutolsó
- Szivárgás
- mint
- Valószínű
- LLM
- csinál
- mód
- kézikönyv
- kézzel
- sok
- tömeges
- gyufa
- említett
- csupán
- modell
- modellek
- több
- többnyire
- közel
- Új
- neves
- NSFW
- számos
- of
- gyakran
- on
- ONE
- csak
- nyitva
- nyílt forráskódú
- OpenAI
- működik
- or
- Más
- Egyéb
- mi
- ki
- Eredmény
- teljesítmény
- kimenetek
- felett
- Papír
- rész
- múlt
- minták
- személyes
- Személyesen
- kifejezés
- Plató
- Platón adatintelligencia
- PlatoData
- Népszerű
- potenciálisan
- pontosan
- magánélet
- gyárt
- Termelés
- Programozás
- utasításokat
- lekérdezések
- Olvasás
- marad
- ismétlés
- jelentést
- kérni
- kutatás
- kutatók
- Reagálni
- kapott
- s
- biztosítékok
- azonos
- skálázható
- Keresés
- küld
- kellene
- előadás
- mutatott
- aláírás
- jelentősen
- Egyszerű
- Méret
- kicsi
- So
- néhány
- kifinomult
- forrás
- Források
- különleges
- Stratégia
- tanulmányok
- Tanulmány
- stílus
- ilyen
- javasolja,
- fogékonyság
- csapat
- technika
- technikák
- teszt
- kipróbált
- szöveg
- mint
- hogy
- A
- azok
- akkor
- Ezek
- ők
- ezt
- Keresztül
- alkalommal
- címmel
- nak nek
- vett
- Vonat
- Képzések
- kiváltó
- Kétszer
- tipikus
- jellemzően
- mögöttes
- megért
- egyedi
- Egyetemek
- egyetemi
- ismeretlen
- USAdollár
- használ
- használt
- használó
- használ
- segítségével
- ellenőrzése
- volt
- Út..
- we
- háló
- voltak
- Mit
- amikor
- ami
- WHO
- akinek
- val vel
- nélkül
- szó
- szavak
- Munka
- érdemes
- lenne
- írt
- év
- Igen
- engedett
- zephyrnet