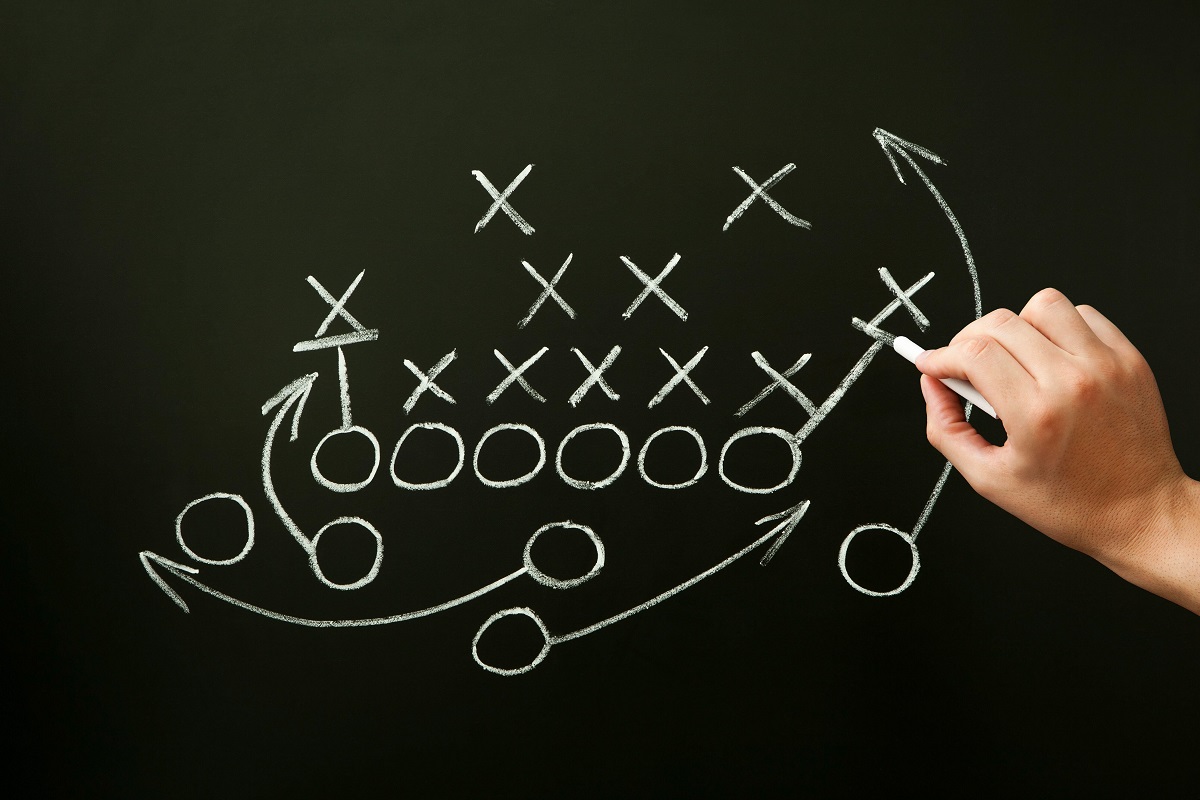
Every company should have a general incident response plan that establishes an incident response team, designates the members, and outlines their strategy for reacting to any cybersecurity incident.
To consistently act on that strategy, however, companies need playbooks — tactical guides that walk responders through investigation, analysis, containment, eradication, and recovery for attacks such as ransomware, a malware outbreak, or business email compromise. Organizations that do not follow a playbook for security will frequently suffer more serious incidents, says John Hollenberger, senior security consultant with Fortinet’s Proactive Services group. In nearly 40% of the global incidents Fortinet handles, the lack of adequate playbooks was a contributing factor that led to the intrusion in the first place.
“Quite often we have found that while the company may have the right tools to detect and respond, there was no, or inadequate, processes around said tools,” Hollenberger says. Even with playbooks, he says, analysts still have complex decisions to make based on the details of the compromise. He adds, “Without knowledge and forethought by an analyst, the wrong approach may be taken or ultimately hinder response efforts.”
Unsurprisingly, companies and researchers are increasingly trying to apply machine learning and artificial intelligence to playbooks — such as getting recommendations on what steps to take while investigating and responding to an incident. A deep neural network can be trained to outperform current heuristic-based schemes, recommending next steps automatically based on the features of an incident and playbooks represented as a series of steps in a graph, according to a paper published in early November by a group of researchers from Ben-Gurion University of the Negev and technology giant NEC.
The BGU and NEC researchers argue that manually managing playbooks can be untenable in the long run.
“Once defined, playbooks are hard-coded for a fixed set of alerts and are fairly static and rigid,” the researchers stated in their paper. “This may be acceptable in the case of investigative playbooks, which may not need to be changed frequently, but it is less desirable in the case of response playbooks, which may need to be changed in order to adapt to emerging threats and novel, previously unseen alerts.”
Proper Reactions Require Playbooks
Automating the detection, investigation, and response to events are the domains of security orchestration, automation, and response (SOAR) systems, which — among other roles — have become the repositories of playbooks to use in the variety of circumstances firms face during a cybersecurity event.
“The world of security is dealing with probabilities and uncertainties — playbooks are a way to reduce further uncertainty by applying a rigorous process to gain predictable final outcomes,” says Josh Blackwelder, deputy chief information security officer at SentinelOne, adding that repeatable outcomes requires the automated application of playbooks through SOAR. “There’s no magical way to go from uncertain security alerts to predictable outcomes without a consistent and logical process flow.”
SOAR systems are becoming increasingly automated, as their name suggests, and adopting AI/ML models to add intelligence to the systems is a natural next step, according to experts.
Managed detection and response firm Red Canary, for example, currently uses AI to identify patterns and trends that are useful in detecting and responding to threats and reducing the cognitive load on analysts to make them more efficient and effective. In addition, generative AI systems can make it easier to communication both a summary and the technical details of incidents to customers, says Keith McCammon, chief security officer and co-founder of Red Canary.
“We don’t use AI to do things like make more playbooks, but we are using it extensively to make execution of playbooks and other security operations processes faster and more effective,” he says.
Eventually, playbooks may be fully automated through deep learning (DL) neural networks, the BGU and NEC researchers wrote. “[W]e aim at extending our method to support complete end-to-end pipeline where, once an alert is received by the SOAR system, a DL-based model handles the alert and deploys appropriate responses automatically — dynamically and autonomously creating on-the-fly playbooks — and thus reducing the burden on security analysts,” they wrote.
Yet giving AI/ML models the ability to manage and update playbooks should be done with care, especially in sensitive or regulated industries, says Andrea Fumagalli, senior director of orchestration and automation for Sumo Logic. The cloud-based security management company uses AI/ML-driven models in its platform and for finding and highlighting threat signals in the data.
“Based on multiple surveys that we’ve conducted with our customers over the years, they are not comfortable yet having AI adapting, amending, and creating playbooks autonomously, either for security reasons or for compliance,” he says. “Enterprise customers want to have full control over what is implemented as incident management and response procedures.”
Automation needs to be fully transparent, and one way to do that is by showing all the queries and data to the security analysts. “This allows the user to sanity-check the logic and data that is returned and validate the results before moving to the next step,” says SentinelOne’s Blackwelder. “We feel this AI-assisted approach is the appropriate balance between the risks of AI and the need to accelerate efficiencies to match the rapidly changing threat landscape.”
- SEO Powered Content & PR Distribution. Get Amplified Today.
- PlatoData.Network Vertical Generative Ai. Empower Yourself. Access Here.
- PlatoAiStream. Web3 Intelligence. Knowledge Amplified. Access Here.
- PlatoESG. Carbon, CleanTech, Energy, Environment, Solar, Waste Management. Access Here.
- PlatoHealth. Biotech and Clinical Trials Intelligence. Access Here.
- Source: https://www.darkreading.com/cybersecurity-operations/automation-via-machine-learning-makes-cybersecurity-playbooks-better
- :is
- :not
- :where
- 7
- a
- ability
- accelerate
- acceptable
- According
- Act
- adapt
- adapting
- add
- adding
- addition
- Adds
- Adopting
- AI
- AI systems
- AI/ML
- aim
- Alert
- Alerts
- All
- allows
- among
- an
- analysis
- analyst
- Analysts
- and
- any
- Application
- Apply
- Applying
- approach
- appropriate
- ARE
- argue
- around
- artificial
- artificial intelligence
- AS
- At
- Attacks
- Automated
- automatically
- Automation
- autonomously
- Balance
- based
- BE
- become
- becoming
- before
- Ben-Gurion University
- between
- both
- burden
- business
- business email compromise
- but
- by
- CAN
- care
- case
- changed
- changing
- chief
- chief information security officer
- circumstances
- Co-founder
- cognitive
- comfortable
- Communication
- Companies
- company
- complete
- complex
- compliance
- compromise
- conducted
- consistent
- consistently
- consultant
- Containment
- contributing
- control
- Creating
- Current
- Currently
- Customers
- Cybersecurity
- data
- dealing
- decisions
- deep
- deep learning
- defined
- deploys
- deputy
- details
- detect
- Detection
- Director
- do
- domains
- don
- done
- during
- dynamically
- Early
- easier
- Effective
- efficiencies
- efficient
- efforts
- either
- emerging
- end-to-end
- enhancing
- Enterprise
- especially
- establishes
- Even
- Event
- events
- example
- execution
- experts
- extending
- extensively
- Face
- factor
- fairly
- faster
- Features
- feel
- final
- finding
- Firm
- firms
- First
- fixed
- flow
- follow
- For
- Fortinet
- found
- frequently
- from
- full
- fully
- further
- Gain
- General
- generative
- Generative AI
- getting
- giant
- Giving
- Global
- Go
- graph
- Group
- Guides
- Handles
- Have
- having
- he
- highlighting
- hinder
- However
- HTTPS
- identify
- implemented
- in
- incident
- incident response
- increasingly
- industries
- information
- information security
- Intelligence
- investigating
- investigation
- investigative
- IT
- ITS
- John
- jpg
- keith
- knowledge
- Lack
- landscape
- learning
- Led
- less
- like
- load
- logic
- logical
- Long
- machine
- machine learning
- make
- malware
- manage
- management
- managing
- manually
- Match
- May..
- Members
- method
- model
- models
- more
- more efficient
- moving
- multiple
- name
- Natural
- nearly
- Need
- needs
- network
- networks
- neural
- neural network
- neural networks
- next
- no
- novel
- of
- Officer
- often
- on
- once
- ONE
- Operations
- or
- orchestration
- order
- organizations
- Other
- our
- outbreak
- outcomes
- outlines
- Outperform
- over
- Paper
- patterns
- pipeline
- Place
- plan
- platform
- plato
- Plato Data Intelligence
- PlatoData
- Predictable
- previously
- Proactive
- procedures
- process
- processes
- published
- queries
- quite
- ransomware
- rapidly
- reactions
- reasons
- received
- recommendations
- recommending
- recovery
- Red
- reduce
- reducing
- regulated
- regulated industries
- repeatable
- represented
- require
- requires
- researchers
- Respond
- responding
- response
- responses
- Results
- right
- rigid
- rigorous
- risks
- roles
- Run
- s
- Said
- says
- schemes
- security
- senior
- sensitive
- Series
- serious
- Services
- set
- should
- signals
- soar
- stated
- static
- Step
- Steps
- Still
- Strategy
- such
- Suggests
- SUMMARY
- support
- system
- Systems
- Take
- taken
- team
- Technical
- Technology
- that
- The
- the world
- their
- Them
- There.
- they
- things
- this
- threat
- threats
- Through
- Thus
- to
- tools
- trained
- transparent
- Trends
- trying
- Ultimately
- Uncertain
- uncertainties
- Uncertainty
- university
- Update
- use
- User
- uses
- using
- VALIDATE
- variety
- Ve
- W
- walk
- want
- was
- Way..
- we
- What
- What is
- which
- while
- will
- with
- without
- world
- Wrong
- wrote
- years
- yet
- zephyrnet