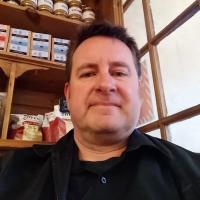
2023 was a troublesome year for many on our planet – wars, violence, population displacement, catastrophes, extremism, higher costs of living and poverty. Folks working in our industry were comparatively lucky, some of us invigorated by the exciting GenerativeAI tornado. Just as HFT transformed the vocabulary of capital markets in the 2000s, and digitalization transformed banking and financial services vocabulary in the 2010s, GenAI has brought us a new AI lexicon, at quite some speed too.
With it, we encountered many terms, many barely used in 2022 but which now carry new or very different meanings. I and many others in Financial Services, use them intraday every day. If you’re one of the (un)lucky few who doesn’t, here’s a quick refresher of my favorite three!
Term 1: The Vector Database
The so-called vector database has become core to many enterprise GenAI stacks, as a means of improving the quality of responses to prompts. The alternatives, e.g., “fine tuning” large language models [LLM] without an accompanying database, are expensive, and fraught with risk and compliance overheads. A vector database captures proprietary enterprise information, brings cost effectiveness, and gives comparative control. Financial services firms are certainly in the queue to use vector databases.
Ironically in finance, vectors have for years been integral to the matrix algebra predominant in trading and risk management. Data storage of such “vectors” and matrices have also been around for decades, typically in columnar databases, or as tables or dataframes used in languages like Python (Pandas), R, MATLAB and SAS. When retrieved and used, for example as financial time-series and panel data, in conjunction with techniques like linear and time-series regressions, they drive predictive analytics, anomaly detection, and econometrics. They also help inform backtesting, most notably of trading, portfolio management and risk strategies. While capital markets – the front and middle office – led the matrix algebra charge, increasingly analytic-centric use cases such as marketing, fraud detection, and digitalization generally took data science – and vectors – across the financial organizations.
I was fascinated, therefore, when a former colleague went to work for a “vector database” start-up in June 2021. His article on
Solving Complex Problems with Vector Databases from pre-ChatGPT March 2022 caught my eye because he highlighted very specific vector types – vector embeddings – encoded easily-searched navigable vectors capturing knowledge from unstructured information such as words, images, etc. When ChatGPT launched later that year, vector stores of such embedding types were elevated to being key vehicles of managing semantic meaning. Most commonly, stores are vector databases, of which
there are now many. Already, they power financial services and capital markets apps, most commonly
natural language processing use cases, e.g., summarizing legal documents and financial reports, or capturing sentiment from social media and news feeds. However, they are also tackling more
involved applications, augmenting trading and risk management insights for example, often alongside traditional statistics and machine learning.
Incidentally, the company my former colleague went to join became a GenAI unicorn, valued at a healthy $750m. Nice work if you can get it!
Term 2: RAG, aka Retrieval Augmented Generation
RAG was barely a word on anyone’s lips in Spring 2023, at least in the capitalized RAG “Retrieval Augmented Generation” sense of the term. Google search stats for the terms accelerated from about July 2023 and by Autumn/Fall, RAG was everywhere, the predominant pipeline approach by which vector databases help tame Large Language Model “stochastic parrots”. On one hand, RAG encapsulates pipelines to provision enterprise data workflows and on the other pragmatically helps financial firms reduce hallucinations and accommodate internal – and external – risk management and AI compliance processes.
There are
many types of RAG pipelines, and they can appear intimidatingly complex. However, think of RAG simply as providing a data pipeline between prompts, your enterprise data, and large language models. To learn more, and see how it affects finance, read my
finextra blog or watch
this great webcast summarizing the risk management opportunities of RAG. If you begin to implement them at any stage, you’ll likely explore “RAG-friendly” environments like LangChain &
LlamaIndex.
Term 3: Hallucinations
I used the term “hallucinations” in my prior section, posing it as a problem solved by RAG, and, in turn, by vector databases. With GenAI, hallucinations are no longer simply triggers of mind-stimulating creativity, such as the drug-inspired Sergeant Pepper’s Lonely Hearts Club Band by the Beatles, or the Beachboys’ Good Vibrations. Neither are they the preserve of shamanic dreaming practised by many peoples, e.g.
eastern Siberian Chukchi peoples, nor physical activities which utilize mind-altering techniques, such as yoga, massage and tantric sex. The word “hallucination” also now applies to the failings of LLMs to navigate information the models don’t have access to, or misuse existing information. It became very obvious very quickly that
ChatGPT, Bard and similar systems were prone to manufactured “hallucinatory” responses, and these brought risk when ill-informed actions ensued.
Here’s the twist. AI investor Marc Andreessen suggests that while most see hallucinations as bugs, they can be helpful as features when AI is used as a cocreator, a suggester, and a guesser. As a brainstorming aid, their made-up guesses can fuel human creativity. Andreessen for example highlights how lawyers use AI’s “made-up” suggestions during case preparation to imagine novel legal strategies. In Financial services, traders on Wall Street are already use generative AI and vector databases to find trading opportunities — to zig when the masses zag.
Whatever you think of GenAI, it has certainly brought us a delightful new lexicon!
- SEO Powered Content & PR Distribution. Get Amplified Today.
- PlatoData.Network Vertical Generative Ai. Empower Yourself. Access Here.
- PlatoAiStream. Web3 Intelligence. Knowledge Amplified. Access Here.
- PlatoESG. Carbon, CleanTech, Energy, Environment, Solar, Waste Management. Access Here.
- PlatoHealth. Biotech and Clinical Trials Intelligence. Access Here.
- Source: https://www.finextra.com/blogposting/25468/three-genai-terms-financial-practitioners-learnt-in-2023?utm_medium=rssfinextra&utm_source=finextrablogs
- :has
- :is
- 1
- 2021
- 2022
- 2023
- a
- About
- accelerated
- access
- accommodate
- across
- actions
- activities
- AI
- Aid
- aka
- alongside
- already
- also
- alternatives
- an
- analytics
- and
- Andreessen
- any
- appear
- applies
- approach
- apps
- ARE
- around
- article
- AS
- At
- augmented
- Backtesting
- BAND
- Banking
- BE
- Beatles
- became
- because
- become
- been
- begin
- being
- between
- Brings
- brought
- bugs
- but
- by
- CAN
- Can Get
- capital
- Capital Markets
- capitalized
- captures
- Capturing
- carry
- case
- cases
- catastrophes
- caught
- certainly
- charge
- ChatGPT
- club
- colleague
- commonly
- company
- comparatively
- complex
- compliance
- conjunction
- control
- Core
- Cost
- Costs
- creativity
- data
- data science
- data storage
- Database
- databases
- day
- decades
- delightful
- Detection
- different
- digitalization
- displacement
- documents
- Doesn’t
- Dont
- drive
- during
- e
- effectiveness
- elevated
- embedding
- encapsulates
- encoded
- ensued
- Enterprise
- environments
- etc
- Every
- every day
- everywhere
- example
- exciting
- existing
- expensive
- explore
- external
- eye
- Favorite
- Features
- few
- finance
- financial
- financial services
- Find
- Finextra
- firms
- For
- Former
- fraud
- fraud detection
- from
- front
- Fuel
- generally
- generative
- Generative AI
- get
- gives
- good
- Google Search
- great
- hand
- Have
- he
- healthy
- help
- helpful
- helps
- HFT
- higher
- Highlighted
- highlights
- his
- How
- However
- HTML
- HTTPS
- human
- i
- if
- images
- imagine
- implement
- improving
- in
- increasingly
- industry
- inform
- information
- insights
- integral
- internal
- investor
- involved
- IT
- join
- jpg
- July
- june
- just
- Key
- knowledge
- language
- Languages
- large
- later
- launched
- Lawyers
- LEARN
- learning
- least
- Led
- Legal
- like
- likely
- living
- LLM
- longer
- machine
- machine learning
- management
- managing
- manufactured
- many
- March
- Marketing
- Markets
- masses
- Matrix
- meaning
- meanings
- means
- Media
- Middle
- misuse
- model
- models
- more
- most
- my
- Natural
- Natural Language Processing
- Navigate
- Neither
- New
- news
- nice
- no
- notably
- novel
- now
- obvious
- of
- Office
- often
- on
- ONE
- opportunities
- or
- organizations
- Other
- Others
- our
- pandas
- panel
- physical
- pipeline
- planet
- plato
- Plato Data Intelligence
- PlatoData
- population
- portfolio
- portfolio management
- Poverty
- power
- Predictive Analytics
- preparation
- Prior
- Problem
- problems
- processes
- processing
- prompts
- proprietary
- providing
- provision
- Python
- quality
- Quick
- quickly
- quite
- R
- Read
- reduce
- Reports
- responses
- Risk
- risk management
- Science
- Search
- Section
- see
- sense
- sentiment
- Services
- Sex
- similar
- simply
- Social
- social media
- some
- specific
- speed
- spring
- Stacks
- Stage
- Start-up
- statistics
- stats
- storage
- stores
- strategies
- street
- such
- Suggests
- Systems
- tackling
- techniques
- term
- terms
- that
- The
- The Matrix
- their
- Them
- There.
- therefore
- These
- they
- think
- this
- three
- to
- too
- took
- tornado
- Traders
- Trading
- traditional
- transformed
- TURN
- twist
- types
- typically
- UN
- unicorn
- us
- use
- used
- utilize
- valued
- Vehicles
- very
- Violence
- Wall
- Wall Street
- was
- Watch
- we
- went
- were
- when
- which
- while
- WHO
- with
- without
- Word
- words
- Work
- workflows
- working
- year
- years
- Yoga
- You
- Your
- youtube
- zephyrnet