1Center for Quantum Computation and Communication Technology, School of Physics, University of Melbourne, Parkville, 3010, VIC, Australia.
2School of Computing and Information Systems, Melbourne School of Engineering, University of Melbourne, Parkville, 3010, VIC, Australia
3Data61, CSIRO, Clayton, 3168, VIC, Australia
Se vam zdi ta članek zanimiv ali želite razpravljati? Zaslišite ali pustite komentar na SciRate.
Minimalizem
Surface code error correction offers a highly promising pathway to achieve scalable fault-tolerant quantum computing. When operated as stabilizer codes, surface code computations consist of a syndrome decoding step where measured stabilizer operators are used to determine appropriate corrections for errors in physical qubits. Decoding algorithms have undergone substantial development, with recent work incorporating machine learning (ML) techniques. Despite promising initial results, the ML-based syndrome decoders are still limited to small scale demonstrations with low latency and are incapable of handling surface codes with boundary conditions and various shapes needed for lattice surgery and braiding. Here, we report the development of an artificial neural network (ANN) based scalable and fast syndrome decoder capable of decoding surface codes of arbitrary shape and size with data qubits suffering from the depolarizing error model. Based on rigorous training over 50 million random quantum error instances, our ANN decoder is shown to work with code distances exceeding 1000 (more than 4 million physical qubits), which is the largest ML-based decoder demonstration to-date. The established ANN decoder demonstrates an execution time in principle independent of code distance, implying that its implementation on dedicated hardware could potentially offer surface code decoding times of O($mu$sec), commensurate with the experimentally realisable qubit coherence times. With the anticipated scale-up of quantum processors within the next decade, their augmentation with a fast and scalable syndrome decoder such as developed in our work is expected to play a decisive role towards experimental implementation of fault-tolerant quantum information processing.
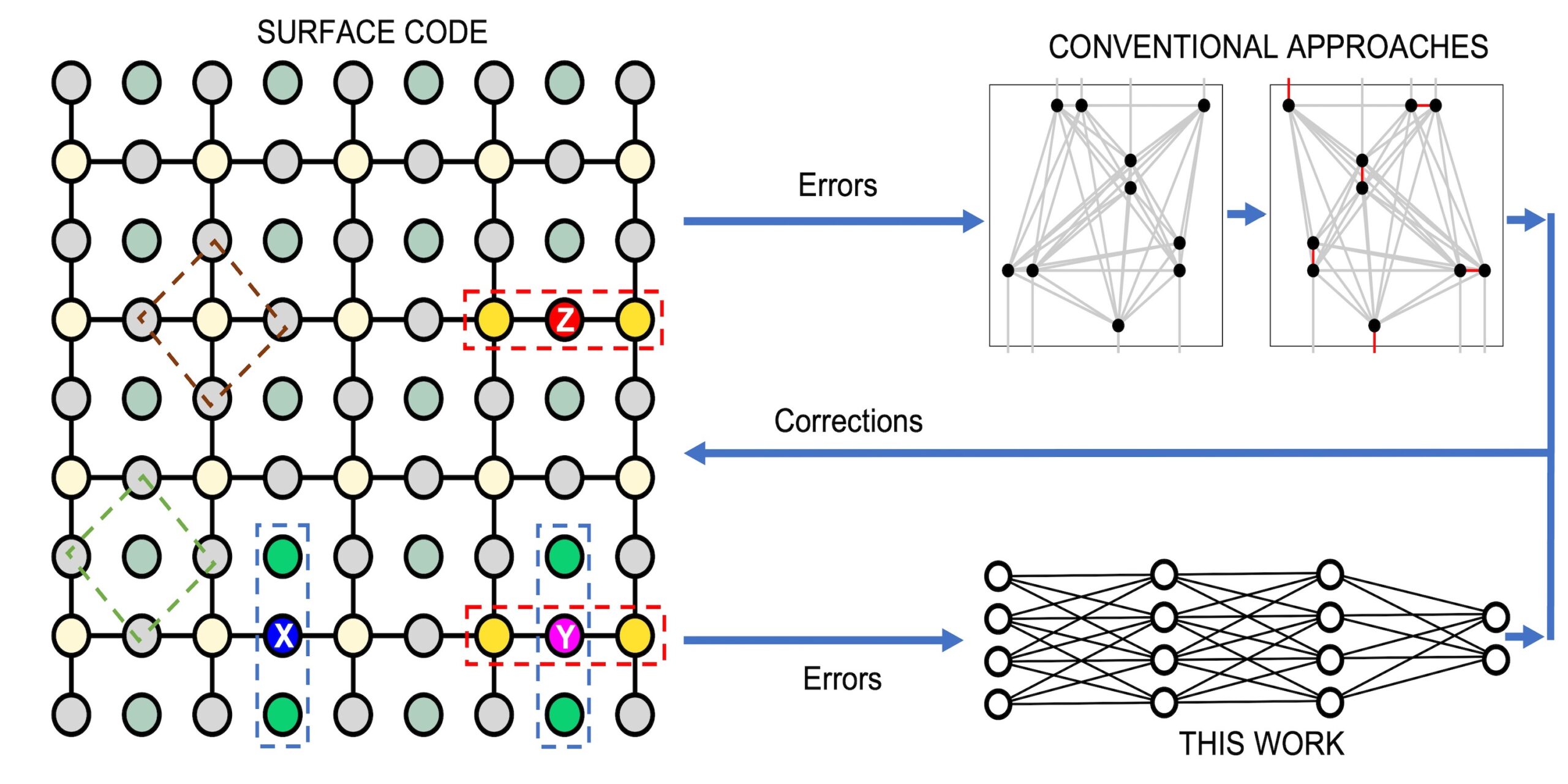
Featured image: Artificial neural network decoder framework for fast and scalable error correction.
Priljubljen povzetek
Our work proposed and implemented a novel convolutional neural network framework to address the scaling problems encountered when decoding large distance surface codes. The convolutional neural network was given an input comprised of changed parity measurements, as well as the boundary structure of the error correction code. Given the finite window of local observation occurring throughout the convolutional neural network, a mop-up decoder was used to correct any sparse residual errors that may remain. Based on rigorous training over 50 million random quantum error instances, our decoder was shown to work with code distances exceeding 1000 (more than 4 million physical qubits), which was the largest ML-based decoder demonstration to-date.
The use of convolutional neural networks and boundary structure in the input allowed our network to be applied on a wide range of surface code distances and boundary configurations. The local connectivity of the network allows low latency to be retained when decoding larger distance codes and readily facilitates parallelization. Our work addresses a key problem in the use of neural networks for decoding at scales of problems of practical interest and allows further research involving the use of networks with similar structure.
► BibTeX podatki
► Reference
[1] S. Pirandola, UL Andersen, L. Banchi, M. Berta, D. Bunandar, R. Colbeck, D. Englund, T. Gehring, C. Lupo, C. Ottaviani, JL Pereira, M. Razavi, J. Shamsul Shaari , M. Tomamichel, VC Usenko, G. Vallone, P. Villoresi in P. Wallden. "Napredek v kvantni kriptografiji". Adv. Opt. Foton. 12, 1012–1236 (2020).
https: / / doi.org/ 10.1364 / AOP.361502
[2] Yudong Cao, Jonathan Romero, Jonathan P. Olson, Matthias Degroote, Peter D. Johnson, Mária Kieferová, Ian D. Kivlichan, Tim Menke, Borja Peropadre, Nicolas PD Sawaya, Sukin Sim, Libor Veis in Alán Aspuru-Guzik. "Kvantna kemija v dobi kvantnega računalništva". Chemical Reviews 119, 10856–10915 (2019).
https: / / doi.org/ 10.1021 / acs.chemrev.8b00803
[3] Román Orús, Samuel Mugel in Enrique Lizaso. "Kvantno računalništvo za finance: pregled in možnosti". Ocene iz fizike 4, 100028 (2019).
https: / / doi.org/ 10.1016 / j.revip.2019.100028
[4] Craig Gidney in Martin Ekerå. »Kako faktorizirati 2048-bitna cela števila RSA v 8 urah z uporabo 20 milijonov hrupnih kubitov«. Quantum 5, 433 (2021).
https://doi.org/10.22331/q-2021-04-15-433
[5] Joonho Lee, Dominic W. Berry, Craig Gidney, William J. Huggins, Jarrod R. McClean, Nathan Wiebe in Ryan Babbush. "Še učinkovitejši kvantni izračuni kemije s tenzorsko hiperkontrakcijo". PRX Quantum 2, 030305 (2021).
https: / / doi.org/ 10.1103 / PRXQuantum.2.030305
[6] Yuval R. Sanders, Dominic W. Berry, Pedro C.S. Costa, Louis W. Tessler, Nathan Wiebe, Craig Gidney, Hartmut Neven, and Ryan Babbush. “Compilation of fault-tolerant quantum heuristics for combinatorial optimization”. PRX Quantum 1, 020312 (2020).
https: / / doi.org/ 10.1103 / PRXQuantum.1.020312
[7] Eric Dennis, Aleksej Kitajev, Andrew Landahl in John Preskill. "Topološki kvantni spomin". Journal of Mathematical Physics 43, 4452–4505 (2002).
https: / / doi.org/ 10.1063 / 1.1499754
[8] Christian Kraglund Andersen, Ants Remm, Stefania Lazar, Sebastian Krinner, Nathan Lacroix, Graham J. Norris, Mihai Gabureac, Christopher Eichler in Andreas Wallraff. "Ponavljajoče se kvantno odkrivanje napak v površinski kodi". Nature Physics 16, 875–880 (2020).
https: / / doi.org/ 10.1038 / s41567-020-0920-y
[9] Zijun Chen, Kevin J Satzinger, Juan Atalaya, Alexander N Korotkov, Andrew Dunsworth, Daniel Sank, Chris Quintana, Matt McEwen, Rami Barends, Paul V Klimov, et al. “Exponential suppression of bit or phase errors with cyclic error correction”. Nature 595, 383–387 (2021).
https: / / doi.org/ 10.1038 / s41586-021-03588-y
[10] Austin G. Fowler, David S. Wang, and Lloyd C. L. Hollenberg. “Surface code quantum error correction incorporating accurate error propagation” (2010). arXiv:1004.0255.
https:///doi.org/10.48550/arXiv.1004.0255
arXiv: 1004.0255
[11] Austin G. Fowler, Adam C. Whiteside in Lloyd CL Hollenberg. “K praktični klasični obdelavi površinske kode”. Physical Review Letters 108 (2012).
https: / / doi.org/ 10.1103 / physrevlett.108.180501
[12] Austin G. Fowler. »Optimalni popravek kompleksnosti koreliranih napak v površinski kodi« (2013). arXiv:1310.0863.
https:///doi.org/10.48550/arXiv.1310.0863
arXiv: 1310.0863
[13] Fern H. E. Watson, Hussain Anwar, and Dan E. Browne. “Fast fault-tolerant decoder for qubit and qudit surface codes”. Phys. Rev. A 92, 032309 (2015).
https: / / doi.org/ 10.1103 / PhysRevA.92.032309
[14] Guillaume Duclos-Cianci and David Poulin. “Fast decoders for topological quantum codes”. Phys. Rev. Lett. 104, 050504 (2010).
https: / / doi.org/ 10.1103 / PhysRevLett.104.050504
[15] Robert Raussendorf in Jim Harrington. "Kvantno računanje, odporno na napake, z visokim pragom v dveh dimenzijah". Phys. Rev. Lett. 98, 190504 (2007).
https: / / doi.org/ 10.1103 / PhysRevLett.98.190504
[16] Daniel Litinski. "Igra površinskih kod: kvantno računalništvo velikega obsega z mrežno kirurgijo". Quantum 3, 128 (2019).
https://doi.org/10.22331/q-2019-03-05-128
[17] Savvas Varsamopoulos, Ben Criger, and Koen Bertels. “Decoding small surface codes with feedforward neural networks”. Quantum Science and Technology 3, 015004 (2017).
https: / / doi.org/ 10.1088 / 2058-9565 / aa955a
[18] Amarsanaa Davaasuren, Yasunari Suzuki, Keisuke Fujii, and Masato Koashi. “General framework for constructing fast and near-optimal machine-learning-based decoder of the topological stabilizer codes”. Phys. Rev. Res. 2, 033399 (2020).
https: / / doi.org/ 10.1103 / PhysRevResearch.2.033399
[19] Giacomo Torlai in Roger G. Melko. "Nevronski dekoder za topološke kode". Phys. Rev. Lett. 119, 030501 (2017).
https: / / doi.org/ 10.1103 / PhysRevLett.119.030501
[20] Stefan Krastanov and Liang Jiang. “Deep neural network probabilistic decoder for stabilizer codes”. Scientific Reports 7 (2017).
https://doi.org/10.1038/s41598-017-11266-1
[21] Paul Baireuther, Thomas E. O'Brien, Brian Tarasinski in Carlo WJ Beenakker. "Popravek koreliranih napak qubit v topološki kodi s pomočjo strojnega učenja". Quantum 2, 48 (2018).
https://doi.org/10.22331/q-2018-01-29-48
[22] Debasmita Bhoumik, Pinaki Sen, Ritajit Majumdar, Susmita Sur-Kolay, Latesh Kumar K J, and Sundaraja Sitharama Iyengar. “Efficient decoding of surface code syndromes for error correction in quantum computing” (2021). arXiv:2110.10896.
https:///doi.org/10.48550/arXiv.2110.10896
arXiv: 2110.10896
[23] Ryan Sweke, Markus S. Kesselring, Evert PL van Nieuwenburg in Jens Eisert. "Dekoderji za okrepljeno učenje za kvantno računanje, odporno na napake". Strojno učenje: znanost in tehnologija 2, 025005 (2020).
https://doi.org/ 10.1088/2632-2153/abc609
[24] Elisha Siddiqui Matekole, Esther Ye, Ramya Iyer, and Samuel Yen-Chi Chen. “Decoding surface codes with deep reinforcement learning and probabilistic policy reuse” (2022). arXiv:2212.11890.
https:///doi.org/10.48550/arXiv.2212.11890
arXiv: 2212.11890
[25] Ramon WJ Overwater, Masoud Babaie in Fabio Sebastiano. »Dekoderji nevronskih omrežij za kvantno odpravljanje napak z uporabo površinskih kod: Vesoljsko raziskovanje kompromisov med stroški in zmogljivostjo strojne opreme«. IEEE Transactions on Quantum Engineering 3, 1–19 (2022).
https: / / doi.org/ 10.1109 / TQE.2022.3174017
[26] Kai Meinerz, Chae-Yeun Park, and Simon Trebst. “Scalable neural decoder for topological surface codes”. Phys. Rev. Lett. 128, 080505 (2022).
https: / / doi.org/ 10.1103 / PhysRevLett.128.080505
[27] S. Varsamopoulos, K. Bertels, and C. Almudever. “Comparing neural network based decoders for the surface code”. IEEE Transactions on Computers 69, 300–311 (2020).
https: / / doi.org/ 10.1109 / TC.2019.2948612
[28] Oscar Higgott. “Pymatching: A python package for decoding quantum codes with minimum-weight perfect matching” (2021). arXiv:2105.13082.
https:///doi.org/10.48550/arXiv.2105.13082
arXiv: 2105.13082
[29] Christopher Chamberland and Pooya Ronagh. “Deep neural decoders for near term fault-tolerant experiments”. Quantum Science and Technology 3, 044002 (2018).
https://doi.org/10.1088/2058-9565/aad1f7
[30] Daniel Gottesman. "Stabilizacijske kode in kvantna korekcija napak" (1997). arXiv:quant-ph/9705052.
https:///doi.org/10.48550/arXiv.quant-ph/9705052
arXiv: kvant-ph / 9705052
[31] Charles D. Hill, Eldad Peretz, Samuel J. Hile, Matthew G. House, Martin Fuechsle, Sven Rogge, Michelle Y. Simmons, and Lloyd C. L. Hollenberg. “A surface code quantum computer in silicon”. Science Advances 1, e1500707 (2015).
https: / / doi.org/ 10.1126 / sciadv.1500707
[32] G. Pica, B. W. Lovett, R. N. Bhatt, T. Schenkel, and S. A. Lyon. “Surface code architecture for donors and dots in silicon with imprecise and nonuniform qubit couplings”. Phys. Rev. B 93, 035306 (2016).
https: / / doi.org/ 10.1103 / PhysRevB.93.035306
[33] Charles D. Hill, Muhammad Usman, and Lloyd C. L. Hollenberg. “An exchange-based surface-code quantum computer architecture in silicon” (2021). arXiv:2107.11981.
https:///doi.org/10.48550/arXiv.2107.11981
arXiv: 2107.11981
[34] Christopher Chamberland, Guanyu Zhu, Theodore J. Yoder, Jared B. Hertzberg in Andrew W. Cross. “Topološke in podsistemske kode na nizkostopenjskih grafih z zastavičnimi kubiti”. Phys. Rev. X 10, 011022 (2020).
https: / / doi.org/ 10.1103 / PhysRevX.10.011022
[35] H. Bombin, Ruben S. Andrist, Masayuki Ohzeki, Helmut G. Katzgraber in MA Martin-Delgado. "Močna odpornost topoloških kod na depolarizacijo". Phys. Rev. X 2, 021004 (2012).
https: / / doi.org/ 10.1103 / PhysRevX.2.021004
[36] Ashley M. Stephens. “Fault-tolerant thresholds for quantum error correction with the surface code”. Phys. Rev. A 89, 022321 (2014).
https: / / doi.org/ 10.1103 / PhysRevA.89.022321
[37] David S. Wang, Austin G. Fowler, and Lloyd C. L. Hollenberg. “Surface code quantum computing with error rates over 1%”. Phys. Rev. A 83, 020302 (2011).
https: / / doi.org/ 10.1103 / PhysRevA.83.020302
[38] Austin G. Fowler in Craig Gidney. »Kvantno računanje z nizkimi stroški z uporabo mrežne kirurgije« (2019). arXiv:1808.06709.
https:///doi.org/10.48550/arXiv.1808.06709
arXiv: 1808.06709
[39] Austin G. Fowler, Matteo Mariantoni, John M. Martinis in Andrew N. Cleland. "Površinske kode: Proti praktičnemu obsežnemu kvantnemu računanju". Physical Review A 86 (2012).
https: / / doi.org/ 10.1103 / physreva.86.032324
[40] Xiaotong Ni. “Neural network decoders for large-distance 2d toric codes”. Quantum 4, 310 (2020).
https://doi.org/10.22331/q-2020-08-24-310
[41] A. Holmes, M. Jokar, G. Pasandi, Y. Ding, M. Pedram, and F. T. Chong. “Nisq+: Boosting quantum computing power by approximating quantum error correction”. In 2020 ACM/IEEE 47th Annual International Symposium on Computer Architecture (ISCA). Pages 556–569. Los Alamitos, CA, USA (2020). IEEE Computer Society.
https:///doi.org/10.1109/ISCA45697.2020.00053
[42] Christian Kraglund Andersen, Ants Remm, Stefania Lazar, Sebastian Krinner, Johannes Heinsoo, Jean-Claude Besse, Mihai Gabureac, Andreas Wallraff, and Christopher Eichler. “Entanglement stabilization using ancilla-based parity detection and real-time feedback in superconducting circuits”. npj Quantum Information 5 (2019).
https://doi.org/10.1038/s41534-019-0185-4
[43] Martín Abadi, Ashish Agarwal, Paul Barham, Eugene Brevdo, Zhifeng Chen, Craig Citro, Greg S. Corrado, Andy Davis, Jeffrey Dean, Matthieu Devin, Sanjay Ghemawat, Ian Goodfellow, Andrew Harp, Geoffrey Irving, Michael Isard, Yangqing Jia, Rafal Jozefowicz, Lukasz Kaiser, Manjunath Kudlur, Josh Levenberg, Dan Mane, Rajat Monga, Sherry Moore, Derek Murray, Chris Olah, Mike Schuster, Jonathon Shlens, Benoit Steiner, Ilya Sutskever, Kunal Talwar, Paul Tucker, Vincent Vanhoucke, Vijay Vasudevan, Fernanda Viegas, Oriol Vinyals, Pete Warden, Martin Wattenberg, Martin Wicke, Yuan Yu, and Xiaoqiang Zheng. “Tensorflow: Large-scale machine learning on heterogeneous distributed systems” (2016). arXiv:1603.04467.
https:///doi.org/10.48550/arXiv.1603.04467
arXiv: 1603.04467
[44] Nicolas Delfosse in Naomi H. Nickerson. “Algoritem za skoraj linearno časovno dekodiranje za topološke kode”. Quantum 5, 595 (2021).
https://doi.org/10.22331/q-2021-12-02-595
[45] Takashi Kobayashi, Joseph Salfi, Cassandra Chua, Joost van der Heijden, Matthew G. House, Dimitrie Culcer, Wayne D. Hutchison, Brett C. Johnson, Jeff C. McCallum, Helge Riemann, Nikolay V. Abrosimov, Peter Becker, Hans-Joachim Pohl, Michelle Y. Simmons, and Sven Rogge. “Engineering long spin coherence times of spin–orbit qubits in silicon”. Nature Materials 20, 38–42 (2020).
https://doi.org/10.1038/s41563-020-0743-3
[46] J. Pablo Bonilla Ataides, David K. Tuckett, Stephen D. Bartlett, Steven T. Flammia, and Benjamin J. Brown. “The XZZX surface code”. Nature Communications 12 (2021).
https://doi.org/10.1038/s41467-021-22274-1
[47] Dmitri E. Nikonov and Ian A. Young. “Benchmarking delay and energy of neural inference circuits”. IEEE Journal on Exploratory Solid-State Computational Devices and Circuits 5, 75–84 (2019).
https://doi.org/10.1109/JXCDC.2019.2956112
[48] Austin G. Fowler. “Minimum weight perfect matching of fault-tolerant topological quantum error correction in average $o(1)$ parallel time” (2014). arXiv:1307.1740.
https:///doi.org/10.48550/arXiv.1307.1740
arXiv: 1307.1740
[49] Vedran Dunjko in Hans J Briegel. »Strojno učenje in umetna inteligenca v kvantni domeni: pregled nedavnega napredka«. Poročila o napredku v fiziki 81, 074001 (2018).
https: / / doi.org/ 10.1088 / 1361-6633 / aab406
[50] Laia Domingo Colomer, Michalis Skotiniotis, and Ramon Muñoz-Tapia. “Reinforcement learning for optimal error correction of toric codes”. Physics Letters A 384, 126353 (2020).
https: / / doi.org/ 10.1016 / j.physleta.2020.126353
[51] Milap Sheth, Sara Zafar Jafarzadeh, and Vlad Gheorghiu. “Neural ensemble decoding for topological quantum error-correcting codes”. Phys. Rev. A 101, 032338 (2020).
https: / / doi.org/ 10.1103 / PhysRevA.101.032338
[52] David Fitzek, Mattias Eliasson, Anton Frisk Kockum, and Mats Granath. “Deep q-learning decoder for depolarizing noise on the toric code”. Phys. Rev. Res. 2, 023230 (2020).
https: / / doi.org/ 10.1103 / PhysRevResearch.2.023230
[53] Savvas Varsamopoulos, Koen Bertels, and Carmen G Almudever. “Decoding surface code with a distributed neural network–based decoder”. Quantum Machine Intelligence 2, 1–12 (2020).
https://doi.org/10.1007/s42484-020-00015-9
[54] Thomas Wagner, Hermann Kampermann, and Dagmar Bruß. “Symmetries for a high-level neural decoder on the toric code”. Phys. Rev. A 102, 042411 (2020).
https: / / doi.org/ 10.1103 / PhysRevA.102.042411
[55] Philip Andreasson, Joel Johansson, Simon Liljestrand in Mats Granath. "Kvantna korekcija napak za torično kodo z uporabo globokega ojačitvenega učenja". Quantum 3, 183 (2019).
https://doi.org/10.22331/q-2019-09-02-183
[56] Nikolas P. Breuckmann and Xiaotong Ni. “Scalable neural network decoders for higher dimensional quantum codes”. Quantum 2, 68 (2018).
https://doi.org/10.22331/q-2018-05-24-68
Navedel
[1] Christopher Chamberland, Luis Goncalves, Prasahnt Sivarajah, Eric Peterson, and Sebastian Grimberg, “Techniques for combining fast local decoders with global decoders under circuit-level noise”, arXiv: 2208.01178, (2022).
[2] Samuel C. Smith, Benjamin J. Brown in Stephen D. Bartlett, "Lokalni predekoder za zmanjšanje pasovne širine in zakasnitve kvantnega odpravljanja napak", Uporabljen fizični pregled 19 3, 034050 (2023).
[3] Xinyu Tan, Fang Zhang, Rui Chao, Yaoyun Shi, and Jianxin Chen, “Scalable surface code decoders with parallelization in time”, arXiv: 2209.09219, (2022).
[4] Maxwell T. West, Sarah M. Erfani, Christopher Leckie, Martin Sevior, Lloyd C. L. Hollenberg, and Muhammad Usman, “Benchmarking adversarially robust quantum machine learning at scale”, Fizični pregled raziskav 5 2, 023186 (2023).
[5] Yosuke Ueno, Masaaki Kondo, Masamitsu Tanaka, Yasunari Suzuki, and Yutaka Tabuchi, “NEO-QEC: Neural Network Enhanced Online Superconducting Decoder for Surface Codes”, arXiv: 2208.05758, (2022).
[6] Mengyu Zhang, Xiangyu Ren, Guanglei Xi, Zhenxing Zhang, Qiaonian Yu, Fuming Liu, Hualiang Zhang, Shengyu Zhang, and Yi-Cong Zheng, “A Scalable, Fast and Programmable Neural Decoder for Fault-Tolerant Quantum Computation Using Surface Codes”, arXiv: 2305.15767, (2023).
[7] Karl Hammar, Alexei Orekhov, Patrik Wallin Hybelius, Anna Katariina Wisakanto, Basudha Srivastava, Anton Frisk Kockum, and Mats Granath, “Error-rate-agnostic decoding of topological stabilizer codes”, Fizični pregled A 105 4, 042616 (2022).
[8] Maxwell T. West and Muhammad Usman, “Framework for Donor-Qubit Spatial Metrology in Silicon with Depths Approaching the Bulk Limit”, Uporabljen fizični pregled 17 2, 024070 (2022).
[9] Maxwell T. West, Shu-Lok Tsang, Jia S. Low, Charles D. Hill, Christopher Leckie, Lloyd C. L. Hollenberg, Sarah M. Erfani, and Muhammad Usman, “Towards quantum enhanced adversarial robustness in machine learning”, arXiv: 2306.12688, (2023).
[10] Moritz Lange, Pontus Havström, Basudha Srivastava, Valdemar Bergentall, Karl Hammar, Olivia Heuts, Evert van Nieuwenburg in Mats Granath, »Data-driven decoding of quantum error correcting codes using graph neural networks«, arXiv: 2307.01241, (2023).
Zgornji citati so iz SAO / NASA ADS (zadnjič posodobljeno 2023-07-12 14:31:13). Seznam je morda nepopoln, saj vsi založniki ne dajejo ustreznih in popolnih podatkov o citiranju.
Pridobitve ni bilo mogoče Crossref citirani podatki med zadnjim poskusom 2023-07-12 14:31:11: Citiranih podatkov za 10.22331 / q-2023-07-12-1058 od Crossrefa ni bilo mogoče pridobiti. To je normalno, če je bil DOI registriran pred kratkim.
Ta dokument je objavljen v Quantumu pod Priznanje avtorstva Creative Commons 4.0 International (CC BY 4.0) licenca. Avtorske pravice ostajajo pri izvirnih imetnikih avtorskih pravic, kot so avtorji ali njihove ustanove.
- Distribucija vsebine in PR s pomočjo SEO. Okrepite se še danes.
- PlatoData.Network Vertical Generative Ai. Opolnomočite se. Dostopite tukaj.
- PlatoAiStream. Web3 Intelligence. Razširjeno znanje. Dostopite tukaj.
- PlatoESG. Avtomobili/EV, Ogljik, CleanTech, Energija, Okolje, sončna energija, Ravnanje z odpadki. Dostopite tukaj.
- BlockOffsets. Posodobitev okoljskega offset lastništva. Dostopite tukaj.
- vir: https://quantum-journal.org/papers/q-2023-07-12-1058/
- :ima
- : je
- :ne
- :kje
- ][str
- 1
- 10
- 11
- 12
- 13
- 14
- 15%
- 16
- 17
- 19
- 20
- 2011
- 2012
- 2013
- 2014
- 2015
- 2016
- 2017
- 2018
- 2019
- 2020
- 2021
- 2022
- 2023
- 22
- 23
- 24
- 25
- 26%
- 27
- 28
- 2D
- 30
- 31
- 32
- 33
- 36
- 39
- 40
- 49
- 50
- 51
- 7
- 8
- 9
- 98
- a
- nad
- POVZETEK
- dostop
- natančnost
- natančna
- Doseči
- doseže
- čez
- aktivna
- Adam
- Naslov
- naslovi
- napredek
- kontradiktorno
- pripadnosti
- starost
- AL
- Alexander
- algoritem
- algoritmi
- vsi
- dovoljene
- omogoča
- Prav tako
- an
- in
- Andrew
- letno
- Predvideno
- kaj
- uporabna
- približuje se
- primerno
- Arhitektura
- SE
- umetni
- Umetna inteligenca
- AS
- At
- Austin
- Avstralija
- Avtor
- Avtorji
- povprečno
- pasovna širina
- temeljijo
- BE
- počutje
- ben
- primerjalna analiza
- Benjamin
- Bit
- povečanje
- Meja
- Break
- Brian
- by
- CA
- izračun
- CAN
- lahko
- povzročilo
- spremenilo
- Charles
- kemijske
- kemija
- chen
- chong
- chris
- Christopher
- Koda
- Kode
- združevanje
- komentar
- Commons
- Komunikacija
- Communications
- dokončanje
- kompleksnost
- Sestavljeno
- računanje
- izračuni
- računalnik
- računalniki
- računalništvo
- računalniška moč
- Pogoji
- Povezovanje
- gradnjo
- avtorske pravice
- popravi
- Popravki
- bi
- Craig
- Cross
- ključnega pomena
- kriptografija
- Trenutna
- Daniel
- datum
- Podatkov usmerjenih
- David
- Davis
- desetletje
- Odločilno
- dekodiranje
- namenjen
- globoko
- zamuda
- dokazuje,
- razporejeni
- Globine
- Derek
- Kljub
- odkrivanje
- Odkrivanje
- Ugotovite,
- razvili
- Razvoj
- naprave
- dimenzije
- neposredno
- razpravlja
- razdalja
- porazdeljena
- porazdeljeni sistemi
- domena
- opravljeno
- med
- e
- E&T
- učinkovito
- učinkovite
- energija
- Inženiring
- okrepljeno
- dovolj
- Napaka
- napake
- ustanovljena
- eugene
- presega
- izvedba
- Pričakuje
- Poskusi
- raziskovanje
- olajša
- Faktor
- FAST
- povratne informacije
- financiranje
- za
- Okvirni
- iz
- nadalje
- igra
- generacija
- dana
- Globalno
- graf
- grafi
- Ravnanje
- strojna oprema
- harvard
- Imajo
- tukaj
- visoka
- na visoki ravni
- več
- zelo
- imetniki
- URE
- Hiša
- HTTPS
- IEEE
- if
- slika
- Izvajanje
- izvajali
- in
- ne more
- vključujoč
- Neodvisni
- Podatki
- Informacijski sistemi
- začetna
- vhod
- Institucije
- Intelligence
- obresti
- Zanimivo
- Facebook Global
- preiskuje
- vključujejo
- ITS
- JavaScript
- jeffrey
- Jim
- John
- Johnson
- Revija
- jpg
- karl
- Ključne
- velika
- obsežne
- večja
- Največji
- Zadnja
- Latenca
- učenje
- pustite
- Lee
- Licenca
- LIMIT
- Limited
- Seznam
- lokalna
- logično
- Long
- Pogledal
- jih
- Louis
- nizka
- stroj
- strojno učenje
- Martin
- ujemanje
- materiali
- matematični
- Matthew
- max širine
- Maxwell
- Maj ..
- mcclean
- meritve
- Melbourne
- Spomin
- Meroslovje
- Michael
- mike
- milijonov
- minimalna
- ML
- Model
- mesec
- več
- učinkovitejše
- Murray
- Narava
- Blizu
- Nimate
- potrebna
- potrebe
- mreža
- omrežij
- nevronska mreža
- nevronske mreže
- Naslednja
- Nicolas
- hrup
- normalno
- roman
- se pojavljajo
- of
- ponudba
- Ponudbe
- on
- na spletu
- odprite
- upravlja
- operaterji
- optimalna
- optimizacija
- or
- Da
- izvirno
- naši
- več
- pregled
- Pace
- paket
- strani
- Papir
- vzporedno
- pariteta
- parkirati
- pot
- paul
- popolna
- Peter
- Peterson
- faza
- fizično
- Fizika
- platon
- Platonova podatkovna inteligenca
- PlatoData
- Predvajaj
- politika
- potencialno
- moč
- Praktično
- prejšnja
- v prvi vrsti
- Načelo
- problem
- Težave
- obravnavati
- procesorji
- Napredek
- obetaven
- predlagano
- možnosti
- zagotavljajo
- objavljeno
- Založnik
- založnikov
- Python
- Kvantna
- Kvantni računalnik
- kvantno računalništvo
- kvantna kriptografija
- kvantno odpravljanje napak
- kvantne informacije
- kvantno strojno učenje
- qubit
- qubits
- RAMI
- naključno
- območje
- hitro
- Cene
- v realnem času
- nedavno
- Pred kratkim
- zmanjša
- reference
- registriranih
- ostajajo
- ostanki
- severnih jelenov
- poročilo
- Poročila
- Raziskave
- odpornost
- Rezultati
- ponovna
- pregleda
- Mnenja
- strog
- ROBERT
- robusten
- robustnost
- vloga
- RSA
- Ryan
- s
- brusilniki
- razširljive
- Lestvica
- povečanje
- luske
- skaliranje
- sheme
- <span style="color: #f7f7f7;">Šola</span>
- Tehniška
- Znanost
- Znanost in tehnologija
- znanstveno
- Oblikujte
- Oblike
- pokazale
- Silicij
- DA
- Podoben
- Simon
- hkrati
- Velikosti
- majhna
- Društvo
- SOLVE
- nekaj
- Vesolje
- prostorsko
- Spin
- Korak
- Stephen
- steven
- Še vedno
- Struktura
- precejšen
- Uspešno
- taka
- trpljenje
- Trpi
- primerna
- superprevodni
- zatiranje
- Površina
- Surgery
- Simpozij
- sistemi
- Naloga
- tehnike
- Tehnologija
- Izraz
- kot
- da
- O
- njihove
- ta
- Prag
- skozi
- vsej
- Tim
- čas
- krat
- Naslov
- do
- topološki kvant
- proti
- usposabljanje
- Transakcije
- dva
- pod
- podvrženi
- osnovni
- univerza
- posodobljeno
- URL
- ZDA
- uporaba
- Rabljeni
- uporablja
- uporabo
- različnih
- vincent
- Vlad
- Obseg
- W
- želeli
- je
- Watson
- we
- teža
- Dobro
- West
- kdaj
- ki
- široka
- Širok spekter
- okno
- z
- v
- delo
- X
- xi
- Ye
- leto
- mladi
- Yuan
- zefirnet