When a customer has a production-ready inteligentna obdelava dokumentov (IDP) workload, we often receive requests for a Well-Architected review. To build an enterprise solution, developer resources, cost, time and user-experience have to be balanced to achieve the desired business outcome. The Dobro zasnovan okvir AWS provides a systematic way for organizations to learn operational and architectural best practices for designing and operating reliable, secure, efficient, cost-effective, and sustainable workloads in the cloud.
The IDP Well-Architected Custom Lens follows the AWS Well-Architected Framework, reviewing the solution with six pillars with the granularity of a specific AI or machine learning (ML) use case, and providing the guidance to tackle common challenges. The IDP Well-Architected Custom Lens in the Dobro zasnovano orodje contains questions regarding each of the pillars. By answering these questions, you can identify potential risks and resolve them by following your improvement plan.
This post focuses on the Performance Efficiency pillar of the IDP workload. We dive deep into designing and implementing the solution to optimize for throughput, latency, and overall performance. We start with discussing some common indicators that you should conduct a Well-Architected review, and introduce the fundamental approaches with design principles. Then we go through each focus area from a technical perspective.
To follow along with this post, you should be familiar with the previous posts in this series (Del 1 in Del 2) and the guidelines in Smernice za inteligentno obdelavo dokumentov na AWS. These resources introduce common AWS services for IDP workloads and suggested workflows. With this knowledge, you’re now ready to learn more about productionizing your workload.
Common indicators
The following are common indicators that you should conduct a Well-Architected Framework review for the Performance Efficiency pillar:
- Visoka latenca – When the latency of optical character recognition (OCR), entity recognition, or the end-to-end workflow takes longer than your previous benchmark, this may be an indicator that the architecture design doesn’t cover load testing or error handling.
- Frequent throttling – You may experience throttling by AWS services like Amazonovo besedilo due to request limits. This means that the architecture needs to be adjusted by reviewing the architecture workflow, synchronous and asynchronous implementation, transactions per second (TPS) calculation, and more.
- Debugging difficulties – When there’s a document process failure, you may not have an effective way to identify where the error is located in the workflow, which service it’s related to, and why the failure occurred. This means the system lacks visibility into logs and failures. Consider revisiting the logging design of the telemetry data and adding infrastructure as code (IaC), such as document processing pipelines, to the solution.
kazalniki | Opis | Architectural Gap |
Visoka zamuda | OCR, entity recognition, or end-to-end workflow latency exceeds previous benchmark |
|
Frequent Throttling | Throttling by AWS services like Amazon Textract due to request limits |
|
Hard to Debug | No visibility into location, cause, and reason for document processing failures |
|
Načela oblikovanja
In this post, we discuss three design principles: delegating complex AI tasks, IaC architectures, and serverless architectures. When you encounter a trade-off between two implementations, you can revisit the design principles with the business priorities of your organization so that you can make decisions effectively.
- Delegating complex AI tasks – You can enable faster AI adoption in your organization by offloading the ML model development lifecycle to managed services and taking advantage of the model development and infrastructure provided by AWS. Rather than requiring your data science and IT teams to build and maintain AI models, you can use pre-trained AI services that can automate tasks for you. This allows your teams to focus on higher-value work that differentiates your business, while the cloud provider handles the complexity of training, deploying, and scaling the AI models.
- IaC architectures – When running an IDP solution, the solution includes multiple AI services to perform the end-to-end workflow chronologically. You can architect the solution with workflow pipelines using Korak funkcije AWS to enhance fault tolerance, parallel processing, visibility, and scalability. These advantages can enable you to optimize the usage and cost of underlying AI services.
- Brez strežnika arhitekture – IDP is often an event-driven solution, initiated by user uploads or scheduled jobs. The solution can be horizontally scaled out by increasing the call rates for the AI services, AWS Lambda, and other services involved. A serverless approach provides scalability without over-provisioning resources, preventing unnecessary expenses. The monitoring behind the serverless design assists in detecting performance issues.
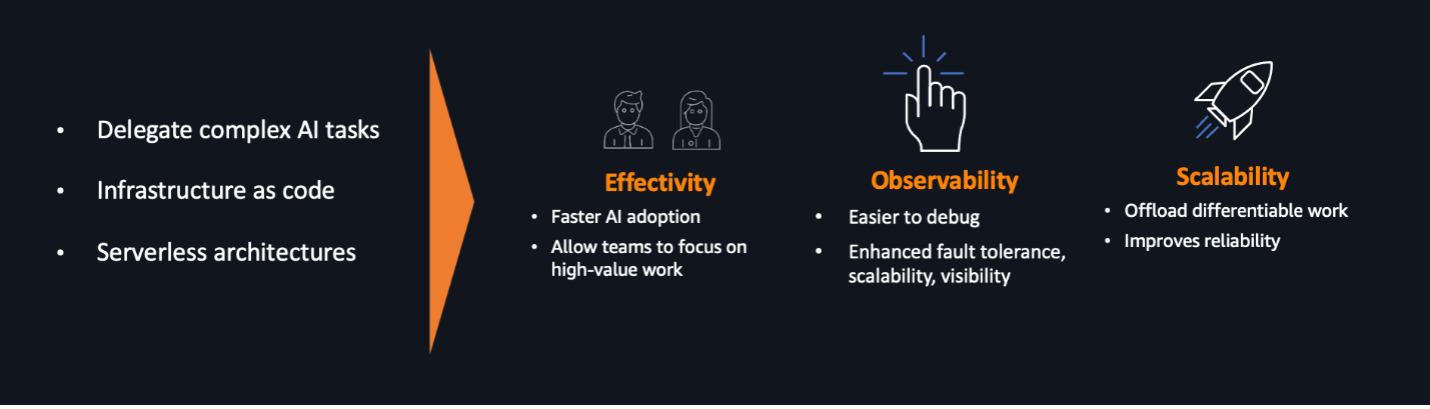
Figure 1.The benefit when applying design principles.
With these three design principles in mind, organizations can establish an effective foundation for AI/ML adoption on cloud platforms. By delegating complexity, implementing resilient infrastructure, and designing for scale, organizations can optimize their AI/ML solutions.
In the following sections, we discuss how to address common challenges in regards to technical focus areas.
Fokusna področja
When reviewing performance efficiency, we review the solution from five focus areas: architecture design, data management, error handling, system monitoring, and model monitoring. With these focus areas, you can conduct an architecture review from different aspects to enhance the effectivity, observability, and scalability of the three components of an AI/ML project, data, model, or business goal.
Arhitekturno oblikovanje
By going through the questions in this focus area, you will review the existing workflow to see if it follows best practices. The suggested workflow provides a common pattern that organizations can follow and prevents trial-and-error costs.
Na podlagi proposed architecture, the workflow follows the six stages of data capture, classification, extraction, enrichment, review and validation, and consumption. In the common indicators we discussed earlier, two out of three come from architecture design problems. This is because when you start a project with an improvised approach, you may meet project restraints when trying to align your infrastructure to your solution. With the architecture design review, the improvised design can be decoupled as stages, and each of them can be reevaluated and reordered.
You can save time, money, and labor by implementing klasifikacije in your workflow, and documents go to downstream applications and APIs based on document type. This enhances the observability of the document process and makes the solution straightforward to maintain when adding new document types.
Upravljanje podatkov
Performance of an IDP solution includes latency, throughput, and the end-to-end user experience. How to manage the document and its extracted information in the solution is the key to data consistency, security, and privacy. Additionally, the solution must handle high data volumes with low latency and high throughput.
When going through the questions of this focus area, you will review the document workflow. This includes data ingestion, data preprocessing, converting documents to document types accepted by Amazon Textract, handling incoming document streams, routing documents by type, and implementing access control and retention policies.
For example, by storing a document in the different processed phases, you can reverse processing to the previous step if needed. The data lifecycle ensures the reliability and compliance for the workload. By using the Amazon Textract Service Quotas Calculator (see the following screenshot), asynchronous features on Amazon Textract, Lambda, Step Functions, Storitev Amazon Simple Queue Service (Amazon SQS) in Amazon Simple notification Service (Amazon SNS), organizations can automate and scale document processing tasks to meet specific workload needs.
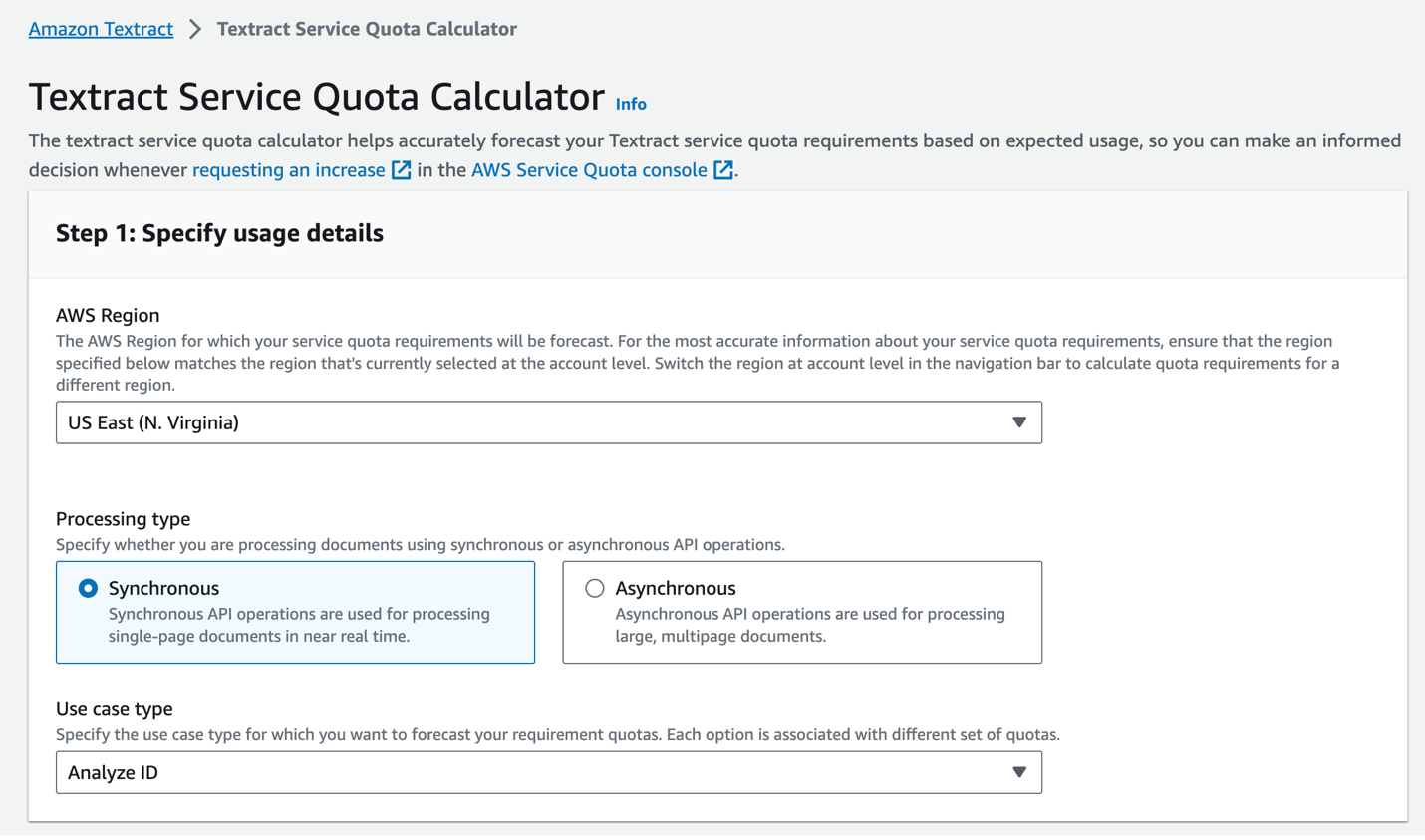
Figure 2. Amazon Textract Service Quota Calculator.
Napaka pri ravnanju
Robust error handling is critical for tracking the document process status, and it provides the operation team time to react to any abnormal behaviors, such as unexpected document volumes, new document types, or other unplanned issues from third-party services. From the organization’s perspective, proper error handling can enhance system uptime and performance.
You can break down error handling into two key aspects:
- AWS service configuration – You can implement retry logic with exponential backoff to handle transient errors like throttling. When you start processing by calling an asynchronous Start* operation, such as StartDocumentTextDetection, you can specify that the completion status of the request is published to an SNS topic in the NotificationChannel configuration. This helps you avoid throttling limits on API calls due to polling the Get* APIs. You can also implement alarms in amazoncloudwatch and triggers to alert when unusual error spikes occur.
- Error report enhancement – This includes detailed messages with an appropriate level of detail by error type and descriptions of error handling responses. With the proper error handling setup, systems can be more resilient by implementing common patterns like automatically retrying intermittent errors, using circuit breakers to handle cascading failures, and monitoring services to gain insight into errors. This allows the solution to balance between retry limits and prevents never-ending circuit loops.
Spremljanje modela
The performance of ML models is monitored for degradation over time. As data and system conditions change, the model performance and efficiency metrics are tracked to ensure retraining is performed when needed.
The ML model in an IDP workflow can be an OCR model, entity recognition model, or classification model. The model can come from an AWS AI service, an open source model on Amazon SageMaker, Amazon Bedrock, or other third-party services. You must understand the limitations and use cases of each service in order to identify ways to improve the model with human feedback and enhance service performance over time.
A common approach is using service logs to understand different levels of accuracy. These logs can help the data science team identify and understand any need for model retraining. Your organization can choose the retraining mechanism—it can be quarterly, monthly, or based on science metrics, such as when accuracy drops below a given threshold.
The goal of monitoring is not just detecting issues, but closing the loop to continuously refine models and keep the IDP solution performing as the external environment evolves.
Nadzor sistema
After you deploy the IDP solution in production, it’s important to monitor key metrics and automation performance to identify areas for improvement. The metrics should include business metrics and technical metrics. This allows the company to evaluate the system’s performance, identify issues, and make improvements to models, rules, and workflows over time to increase the automation rate to understand the operational impact.
On the business side, metrics like extraction accuracy for important fields, overall automation rate indicating the percentage of documents processed without human intervention, and average processing time per document are paramount. These business metrics help quantify the end-user experience and operational efficiency gains.
Technical metrics including error and exception rates occurring throughout the workflow are essential to track from an engineering perspective. The technical metrics can also monitor at each level from end to end and provide a comprehensive view of a complex workload. You can break the metrics down into different levels, such as solution level, end-to-end workflow level, document type level, document level, entity recognition level, and OCR level.
Now that you have reviewed all the questions in this pillar, you can assess the other pillars and develop an improvement plan for your IDP workload.
zaključek
In this post, we discussed common indicators that you may need to perform a Well-Architected Framework review for the Performance Efficiency pillar for your IDP workload. We then walked through design principles to provide a high-level overview and discuss the solution goal. By following these suggestions in reference to the IDP Well-Architected Custom Lens and by reviewing the questions by focus area, you should now have a project improvement plan.
O avtorjih
Mia Chang je specialist za rešitve ML za Amazon Web Services. Dela s strankami v EMEA in deli najboljše prakse za izvajanje delovnih obremenitev AI/ML v oblaku s svojim znanjem iz uporabne matematike, računalništva in AI/ML. Osredotoča se na delovne obremenitve, specifične za NLP, in deli svoje izkušnje kot predavateljica na konferencah in avtorica knjig. V prostem času uživa v pohodništvu, družabnih igrah in kuhanju kave.
Briješ pati je arhitekt za podjetniške rešitve pri AWS. Njegov glavni poudarek je pomagati poslovnim strankam, da za svoje delovne obremenitve sprejmejo tehnologije v oblaku. Ima izkušnje z razvojem aplikacij in arhitekturo podjetja ter je sodeloval s strankami iz različnih panog, kot so šport, finance, energetika in strokovne storitve. Njegovi interesi vključujejo brezstrežniške arhitekture in AI/ML.
Rui Cardoso je partnerski arhitekt rešitev pri Amazon Web Services (AWS). Osredotoča se na AI/ML in IoT. Sodeluje s partnerji AWS in jih podpira pri razvoju rešitev v AWS. Ko ni v službi, uživa v kolesarjenju, pohodništvu in učenju novih stvari.
Tim Condello je višji arhitekt za rešitve za umetno inteligenco (AI) in strojno učenje (ML) pri Amazon Web Services (AWS). Njegov fokus je obdelava naravnega jezika in računalniški vid. Tim uživa v sprejemanju idej strank in njihovem spreminjanju v prilagodljive rešitve.
Sherry Ding je višji arhitekt za rešitve za umetno inteligenco (AI) in strojno učenje (ML) pri Amazon Web Services (AWS). Ima bogate izkušnje s strojnim učenjem z doktoratom iz računalništva. V glavnem dela s strankami iz javnega sektorja pri različnih poslovnih izzivih, povezanih z AI/ML, in jim pomaga pospešiti njihovo pot strojnega učenja v oblaku AWS. Ko ne pomaga strankam, uživa v dejavnostih na prostem.
Suyin Wang je specialist za rešitve AI/ML pri AWS. Ima interdisciplinarno izobrazbo na področju strojnega učenja, finančnih informacijskih storitev in ekonomije, skupaj z dolgoletnimi izkušnjami pri izdelavi aplikacij za podatkovno znanost in strojno učenje, ki so reševale resnične poslovne probleme. Uživa v tem, da strankam pomaga prepoznati prava poslovna vprašanja in gradi prave rešitve AI/ML. V prostem času rada poje in kuha.
- Distribucija vsebine in PR s pomočjo SEO. Okrepite se še danes.
- PlatoData.Network Vertical Generative Ai. Opolnomočite se. Dostopite tukaj.
- PlatoAiStream. Web3 Intelligence. Razširjeno znanje. Dostopite tukaj.
- PlatoESG. Ogljik, CleanTech, Energija, Okolje, sončna energija, Ravnanje z odpadki. Dostopite tukaj.
- PlatoHealth. Obveščanje o biotehnologiji in kliničnih preskušanjih. Dostopite tukaj.
- vir: https://aws.amazon.com/blogs/machine-learning/build-well-architected-idp-solutions-with-a-custom-lens-part-4-performance-efficiency/
- :ima
- : je
- :ne
- :kje
- 1
- 10
- 100
- 32
- 7
- 8
- a
- O meni
- pospeši
- sprejeta
- dostop
- natančnost
- Doseči
- dejavnosti
- dodajanje
- Poleg tega
- Naslov
- Prilagojen
- sprejme
- Sprejetje
- Prednost
- Prednosti
- AI
- AI modeli
- Storitve AI
- AI / ML
- Opozorite
- uskladiti
- vsi
- omogoča
- skupaj
- Prav tako
- Amazon
- Amazonovo besedilo
- Amazon Web Services
- Amazonske spletne storitve (AWS)
- an
- in
- in infrastrukturo
- kaj
- API
- API-ji
- uporaba
- Razvoj aplikacij
- aplikacije
- uporabna
- Uporaba
- pristop
- pristopi
- primerno
- architectural
- Arhitektura
- SE
- OBMOČJE
- območja
- umetni
- Umetna inteligenca
- Umetna inteligenca (AI)
- AS
- vidiki
- oceniti
- pomaga
- At
- Avtor
- avtomatizirati
- samodejno
- Avtomatizacija
- povprečno
- izogniti
- AWS
- ozadje
- Ravnovesje
- uravnotežen
- temeljijo
- BE
- ker
- vedenja
- zadaj
- spodaj
- merilo
- koristi
- BEST
- najboljše prakse
- med
- svet
- Namizne igre
- Knjiga
- Break
- izgradnjo
- Building
- poslovni
- vendar
- by
- izračun
- klic
- kliče
- poziva
- CAN
- zajemanje
- primeru
- primeri
- Vzrok
- izzivi
- spremenite
- značaja
- prepoznavanje znakov
- Izberite
- Razvrstitev
- zapiranje
- Cloud
- Koda
- Kava
- kako
- Skupno
- podjetje
- dokončanje
- kompleksna
- kompleksnost
- skladnost
- deli
- celovito
- računalnik
- Računalništvo
- Računalniška vizija
- Pogoji
- Ravnanje
- Konferenca
- konfiguracija
- Razmislite
- poraba
- Vsebuje
- stalno
- nadzor
- pretvorbo
- strošek
- stroškovno učinkovito
- stroški
- pokrov
- kritično
- po meri
- stranka
- Stranke, ki so
- datum
- Upravljanje podatkov
- znanost o podatkih
- odločitve
- nevezana
- globoko
- Stopnja
- razporedi
- uvajanja
- Oblikovanje
- Načela oblikovanja
- oblikovanje
- želeno
- Podatki
- podrobno
- Razvoj
- Razvojni
- razvoju
- Razvoj
- drugačen
- Težave
- razpravlja
- razpravljali
- razpravljali
- potop
- dokument
- proces dokumentiranja
- Dokumenti
- Ne
- navzdol
- Kapljice
- 2
- vsak
- prej
- Economics
- Izobraževanje
- Učinkovito
- učinkovito
- učinkovitosti
- učinkovite
- EMEA
- omogočajo
- konec
- konec koncev
- energija
- Inženiring
- okrepi
- Izboljša
- obogatitev
- zagotovitev
- zagotavlja
- Podjetje
- entiteta
- okolje
- Napaka
- napake
- bistvena
- vzpostaviti
- oceniti
- razvija
- Primer
- presega
- izjema
- obstoječih
- Stroški
- izkušnje
- eksponentna
- obsežen
- Obširne izkušnje
- zunanja
- pridobivanje
- Napaka
- napake
- seznanjeni
- hitreje
- Lastnosti
- povratne informacije
- Področja
- Slika
- financiranje
- finančna
- finančne informacije
- pet
- Osredotočite
- Osredotoča
- osredotoča
- sledi
- po
- sledi
- za
- Fundacija
- Okvirni
- brezplačno
- iz
- funkcije
- temeljna
- Gain
- zaslužek
- Games
- dana
- Go
- Cilj
- dogaja
- Navodila
- Smernice
- ročaj
- Ročaji
- Ravnanje
- Imajo
- he
- pomoč
- pomoč
- Pomaga
- jo
- visoka
- na visoki ravni
- njegov
- vodoravno
- Kako
- Kako
- HTML
- http
- HTTPS
- človeškega
- Ideje
- identificirati
- if
- vpliv
- izvajati
- Izvajanje
- izvedbe
- izvajanja
- Pomembno
- izboljšanje
- Izboljšanje
- Izboljšave
- in
- vključujejo
- vključuje
- Vključno
- Dohodni
- Povečajte
- narašča
- Kazalec
- kazalniki
- industrij
- Podatki
- Infrastruktura
- začeti
- vpogled
- Intelligence
- Inteligentna
- Inteligentna obdelava dokumentov
- interesi
- intervencije
- v
- uvesti
- vključeni
- Internet stvari
- Vprašanja
- IT
- ITS
- Delovna mesta
- Potovanje
- jpg
- samo
- Imejte
- Ključne
- znanje
- dela
- jezik
- Latenca
- UČITE
- učenje
- Stopnja
- ravni
- življenski krog
- kot
- omejitve
- Meje
- obremenitev
- nahaja
- kraj aktivnosti
- sečnja
- Logika
- več
- ljubi
- nizka
- stroj
- strojno učenje
- v glavnem
- vzdrževati
- Znamka
- IZDELA
- upravljanje
- upravlja
- upravljanje
- matematika
- Maj ..
- pomeni
- Srečati
- sporočil
- Meritve
- moti
- ML
- Model
- modeli
- Denar
- monitor
- spremljati
- spremljanje
- mesečno
- več
- več
- morajo
- naravna
- Obdelava Natural Language
- Nimate
- potrebna
- potrebe
- Novo
- Obvestilo
- zdaj
- zgodilo
- se pojavljajo
- OCR
- of
- pogosto
- on
- odprite
- open source
- deluje
- Delovanje
- operativno
- optično prepoznavanje znakov
- Optimizirajte
- or
- Da
- Organizacija
- organizacije
- Ostalo
- ven
- Rezultat
- več
- Splošni
- pregled
- vzporedno
- Paramount
- del
- partner
- partnerji
- Vzorec
- vzorci
- za
- odstotek
- Izvedite
- performance
- opravljeno
- izvajati
- perspektiva
- Dr.
- steber
- stebri
- Načrt
- Platforme
- platon
- Platonova podatkovna inteligenca
- PlatoData
- politike
- Prispevek
- Prispevkov
- potencial
- vaje
- preprečevanje
- preprečuje
- prejšnja
- primarni
- Načela
- zasebnost
- Težave
- Postopek
- Predelano
- obravnavati
- proizvodnja
- strokovni
- Projekt
- pravilno
- zagotavljajo
- če
- Ponudnik
- zagotavlja
- zagotavljanje
- javnega
- objavljeno
- vprašanja
- Oceniti
- Cene
- precej
- Reagirajo
- pripravljen
- resnični svet
- Razlog
- prejeti
- Priznanje
- reference
- izboljšati
- o
- pozdrav
- povezane
- zanesljivost
- zanesljiv
- poročilo
- zahteva
- zahteva
- odporno
- reševanje
- viri
- odgovorov
- zadrževanje
- nazaj
- pregleda
- Pregledal
- pregledovanje
- Pravica
- tveganja
- usmerjanje
- pravila
- tek
- Shrani
- Prilagodljivost
- razširljive
- Lestvica
- skaliranje
- načrtovano
- Načrtovana delovna mesta
- Znanost
- drugi
- oddelki
- sektor
- zavarovanje
- varnost
- glej
- višji
- Serija
- Brez strežnika
- Storitev
- Storitve
- nastavitev
- Delnice
- je
- shouldnt
- strani
- Enostavno
- SIX
- So
- Rešitev
- rešitve
- nekaj
- vir
- Zvočniki
- specialist
- specifična
- konice
- Šport
- postopka
- Začetek
- Status
- Korak
- shranjevanje
- naravnost
- tokovi
- taka
- podpora
- trajnostno
- sistem
- sistemi
- reševanje
- meni
- ob
- Naloge
- skupina
- Skupine
- tehnični
- Tehnologije
- Testiranje
- kot
- da
- O
- njihove
- Njih
- POTEM
- te
- stvari
- tretjih oseb
- ta
- 3
- Prag
- skozi
- vsej
- pretočnost
- Tim
- čas
- do
- toleranca
- temo
- tps
- sledenje
- Sledenje
- usposabljanje
- Transakcije
- poskuša
- Obračalni
- dva
- tip
- Vrste
- osnovni
- razumeli
- Nepričakovana
- nepotrebna
- uptime
- Uporaba
- uporaba
- primeru uporabe
- uporabnik
- Uporabniška izkušnja
- uporabo
- potrjevanje
- različnih
- Poglej
- vidljivost
- Vizija
- prostornine
- vs
- hodil
- način..
- načini
- we
- web
- spletne storitve
- kdaj
- ki
- medtem
- zakaj
- bo
- z
- brez
- delo
- delal
- potek dela
- delovnih tokov
- deluje
- deluje
- let
- Vi
- Vaša rutina za
- zefirnet