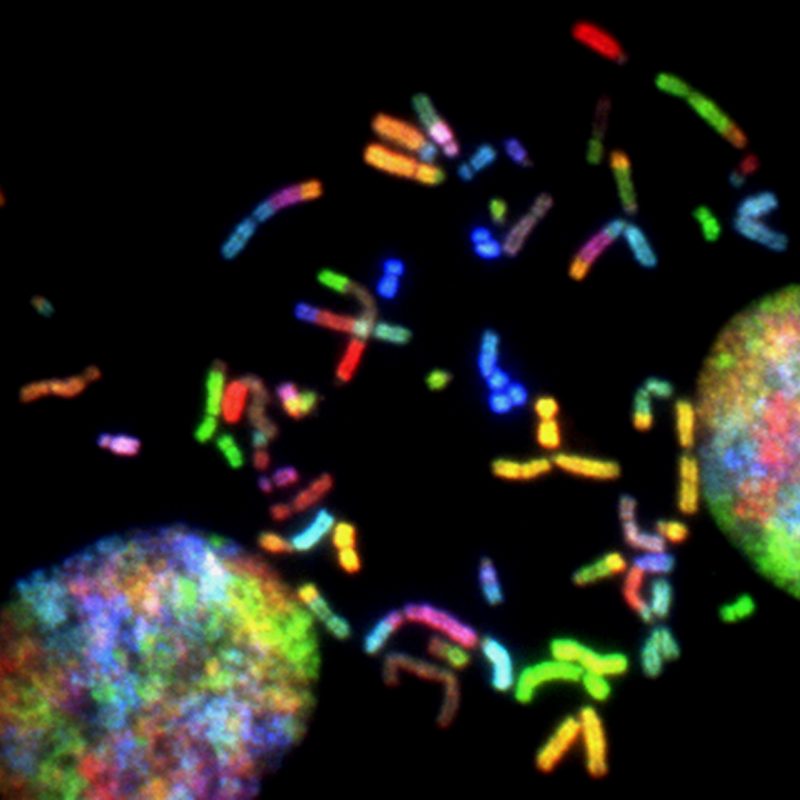
A new Nature Quantum Information paper examines how quantum computing influences gene regulation. Gene Regulatory Networks (GRNs) are crucial for understanding the regulatory relationships between genes in biological systems. These networks help study transcriptional regulation and the molecular basis of regulatory mechanisms, which are crucial for comprehending gene functions in cellular activities. Represented as graphs, GRNs illustrate the interactions between transcription factors and their targets. Single-cell technologies, particularly single-cell RNA sequencing (scRNA-seq), have significantly advanced our ability to study biology at an unprecedented scale and resolution. These technologies measure gene expression in thousands of cells, providing a wealth of data for constructing more precise GRNs. However, traditional computational methods, relying on statistical approaches like correlation, regression, and Bayesian networks, have limitations, especially in capturing simultaneous, inter-regulatory connections between all genes.
Quantum Computing in Biology and GRN Modeling:
Quantum computing, recognized for its potential in various fields, offers a novel approach to modeling GRNs. Quantum algorithms can potentially outperform classical methods in specific computations by leveraging superposition and entanglement phenomena. Introducing a quantum single-cell GRN (qscGRN) modeling method significantly advances this domain. This method uses a parameterized quantum circuit framework for inferring biological GRNs from scRNA-seq data. In the qscGRN model, each gene is represented by a qubit. The model comprises an encoder layer, which translates scRNA-seq data into a superposition state, and regulation layers that entangle qubits to simulate gene-gene interactions. By mapping gene expression values onto a large Hilbert space, the qscGRN model effectively utilizes the information from individual cells to map regulatory relationships.
Application and Potential of Quantum GRN Modeling:
The quantum-classical framework used in this approach includes optimization techniques such as Laplace smoothing and gradient descent algorithms to fine-tune the model parameters. Applied to real scRNA-seq datasets, this method has demonstrated its capability to model gene regulatory relationships effectively, with the network recovered from the quantum circuit showing consistency with previously published GRNs. The successful application of this model to human lymphoblastoid cells, focusing on genes involved in innate immunity regulation, illustrates its potential. The model not only predicted regulatory interactions between genes but also estimated the strength of these interactions.
Future Implications and Research Directions for Gene Regulation:
The integration of quantum computing in biology, specifically in GRN modeling, shows promise in surpassing the limitations of conventional statistical methods. This method offers a deeper understanding of single-cell GRNs by efficiently approaching interconnected genes’ relationships. The findings encourage further exploration into creating quantum algorithms utilizing single-cell data, signaling a new frontier in the intersection of quantum computing and biology. This breakthrough paves the way for future research and could revolutionize our approach to understanding complex biological systems at the molecular level.
Kenna Hughes-Castleberry is the Managing Editor at Inside Quantum Technology and the Science Communicator at JILA (a partnership between the University of Colorado Boulder and NIST). Her writing beats include deep tech, quantum computing, and AI. Her work has been featured in Scientific American, Discover Magazine, New Scientist, Ars Technica, and more.
- SEO Powered Content & PR Distribution. Get Amplified Today.
- PlatoData.Network Vertical Generative Ai. Empower Yourself. Access Here.
- PlatoAiStream. Web3 Intelligence. Knowledge Amplified. Access Here.
- PlatoESG. Carbon, CleanTech, Energy, Environment, Solar, Waste Management. Access Here.
- PlatoHealth. Biotech and Clinical Trials Intelligence. Access Here.
- Source: https://www.insidequantumtechnology.com/news-archive/looking-at-quantum-computing-in-deciphering-gene-regulatory-networks-from-single-cell-data/
- :has
- :is
- :not
- 2023
- 28
- 7
- a
- ability
- activities
- advanced
- advances
- affected
- AI
- algorithms
- All
- also
- American
- an
- and
- Application
- applied
- approach
- approaches
- approaching
- ARE
- AS
- At
- basis
- Bayesian
- BE
- been
- between
- biology
- breakthrough
- but
- by
- CAN
- capability
- Capturing
- Cells
- Colorado
- complex
- comprises
- computational
- computations
- computing
- Connections
- constructing
- conventional
- Correlation
- could
- Creating
- crucial
- data
- datasets
- deep
- deeper
- demonstrated
- discover
- domain
- each
- editor
- effectively
- efficiently
- encourage
- entanglement
- especially
- estimated
- Examines
- exploration
- expression
- factors
- featured
- Fields
- findings
- focusing
- For
- Framework
- from
- Frontier
- functions
- further
- future
- graphs
- harvard
- Have
- help
- her
- High
- How
- However
- HTTPS
- human
- illustrate
- illustrates
- image
- immunity
- implications
- in
- include
- includes
- individual
- information
- innate
- inside
- Inside Quantum Technology
- integration
- interactions
- interconnected
- intersection
- into
- introducing
- involved
- ITS
- jpg
- large
- layer
- layers
- Level
- leveraging
- like
- limitations
- looking
- LOOKS
- magazine
- managing
- map
- mapping
- max-width
- measure
- mechanisms
- method
- methods
- model
- modeling
- molecular
- more
- Nature
- network
- networks
- New
- nist
- nov
- novel
- of
- Offers
- on
- only
- onto
- optimization
- our
- Outperform
- parameters
- particularly
- Partnership
- paves
- plato
- Plato Data Intelligence
- PlatoData
- posted
- potential
- potentially
- precise
- predicted
- previously
- promise
- providing
- published
- Quantum
- quantum algorithms
- quantum computing
- quantum information
- quantum technology
- Qubit
- qubits
- real
- recognized
- Regulation
- regulatory
- Relationships
- relying
- represented
- research
- Resolution
- revolutionize
- RNA
- Scale
- Science
- scientific
- Scientist
- sequencing
- Shows
- significantly
- Space
- specific
- specifically
- statistical
- strength
- Study
- successful
- such
- superposition
- surpassing
- Systems
- targets
- tech
- techniques
- Technologies
- Technology
- that
- The
- the information
- their
- These
- this
- thousands
- to
- traditional
- true
- understanding
- university
- unprecedented
- used
- uses
- utilizes
- Utilizing
- Values
- various
- Way..
- Wealth
- which
- with
- Work
- writing
- zephyrnet