今天我们很高兴地宣布 骆驼守卫 型号现已可供客户使用 亚马逊SageMaker JumpStart。 Llama Guard 在大型语言模型 (LLM) 部署中提供输入和输出保护。它是 Meta 的 Purple Llama 计划的组成部分之一,该计划以开放信任和安全工具及评估为特色,帮助开发人员负责任地使用人工智能模型进行构建。 Purple Llama 汇集了工具和评估,帮助社区负责任地构建生成式 AI 模型。初始版本重点关注网络安全和法学硕士输入和输出保障。 Purple Llama 项目中的组件(包括 Llama Guard 模型)均获得许可,可用于研究和商业用途。
现在,您可以在 SageMaker JumpStart 中使用 Llama Guard 模型。 SageMaker JumpStart 是机器学习 (ML) 中心 亚马逊SageMaker 除了内置算法和端到端解决方案模板之外,它还提供对基础模型的访问,以帮助您快速开始使用 ML。
在这篇文章中,我们将介绍如何部署 Llama Guard 模型并构建负责任的生成式 AI 解决方案。
美洲驼守卫模型
Llama Guard 是 Meta 的一个新模型,为 LLM 部署提供输入和输出护栏。 Llama Guard 是一个公开可用的模型,它在常见的开放基准测试中具有竞争力,并为开发人员提供了预训练模型,以帮助防范生成潜在风险的输出。该模型已经在公开可用的数据集上进行了训练,以便能够检测可能与许多开发人员用例相关的常见类型的潜在风险或违规内容。最终,该模型的愿景是使开发人员能够自定义该模型以支持相关用例,并轻松采用最佳实践并改进开放生态系统。
Llama Guard 可以用作开发人员的补充工具,将其集成到他们自己的缓解策略中,例如聊天机器人、内容审核、客户服务、社交媒体监控和教育。通过在发布或响应之前将用户生成的内容传递给 Llama Guard,开发人员可以标记不安全或不适当的语言,并采取措施维护安全和尊重的环境。
让我们探讨如何在 SageMaker JumpStart 中使用 Llama Guard 模型。
SageMaker 中的基础模型
SageMaker JumpStart 提供对流行模型中心的一系列模型的访问,包括 Hugging Face、PyTorch Hub 和 TensorFlow Hub,您可以在 SageMaker 的 ML 开发工作流程中使用这些模型。 机器学习的最新进展催生了一类新的模型,称为 基础模型,它们通常经过数十亿个参数的训练,并且适用于广泛的用例,例如文本摘要、数字艺术生成和语言翻译。由于这些模型的训练成本很高,因此客户希望使用现有的预先训练的基础模型并根据需要对其进行微调,而不是自己训练这些模型。 SageMaker 提供了精选的模型列表,您可以在 SageMaker 控制台上进行选择。
现在,您可以在 SageMaker JumpStart 中找到来自不同模型提供商的基础模型,从而使您能够快速开始使用基础模型。您可以根据不同的任务或模型提供者找到基础模型,并轻松查看模型特征和使用条款。您还可以使用测试 UI 小部件来尝试这些模型。当您想要大规模使用基础模型时,您可以使用模型提供商提供的预构建笔记本轻松完成此操作,而无需离开 SageMaker。由于模型在 AWS 上托管和部署,因此您可以放心,无论是用于评估还是大规模使用模型,您的数据都不会与第三方共享。
让我们探讨如何在 SageMaker JumpStart 中使用 Llama Guard 模型。
在 SageMaker JumpStart 中探索 Llama Guard 模型
您可以通过 SageMaker Studio UI 和 SageMaker Python SDK 中的 SageMaker JumpStart 访问 Code Llama 基础模型。在本节中,我们将介绍如何发现模型 亚马逊SageMaker Studio.
SageMaker Studio 是一个集成开发环境 (IDE),提供基于 Web 的单一可视化界面,您可以在其中访问专用工具来执行所有 ML 开发步骤,从准备数据到构建、训练和部署 ML 模型。 有关如何开始和设置 SageMaker Studio 的更多详细信息,请参阅 亚马逊SageMaker Studio.
在 SageMaker Studio 中,您可以访问 SageMaker JumpStart,其中包含预训练的模型、笔记本和预构建的解决方案,位于 预构建和自动化解决方案.
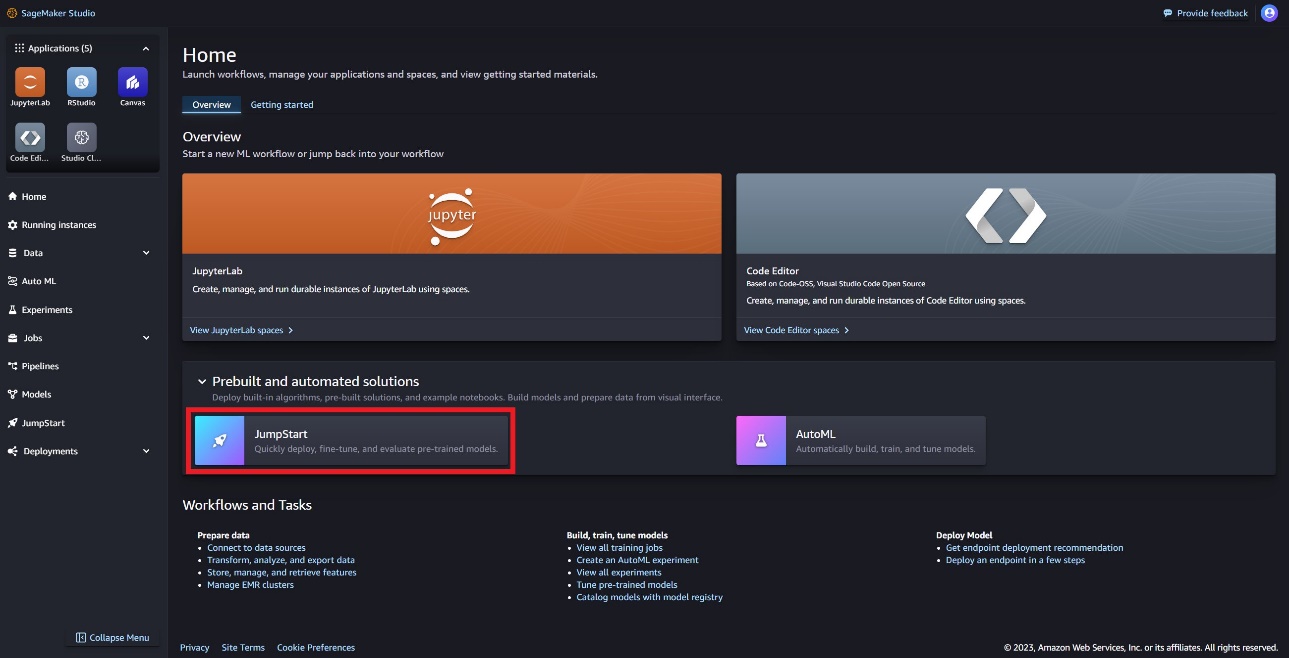
在 SageMaker JumpStart 登录页面上,您可以通过选择 Meta 中心或搜索 Llama Guard 来找到 Llama Guard 模型。
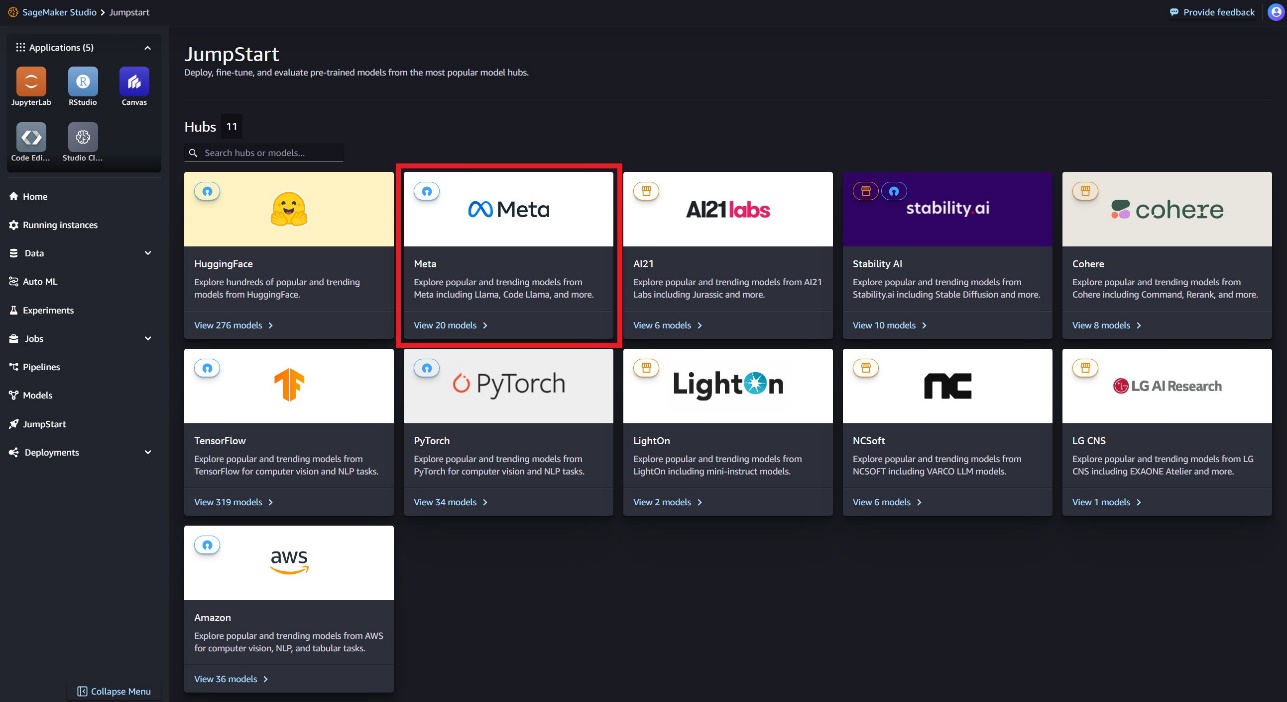
您可以从各种 Llama 模型变体中进行选择,包括 Llama Guard、Llama-2 和 Code Llama。
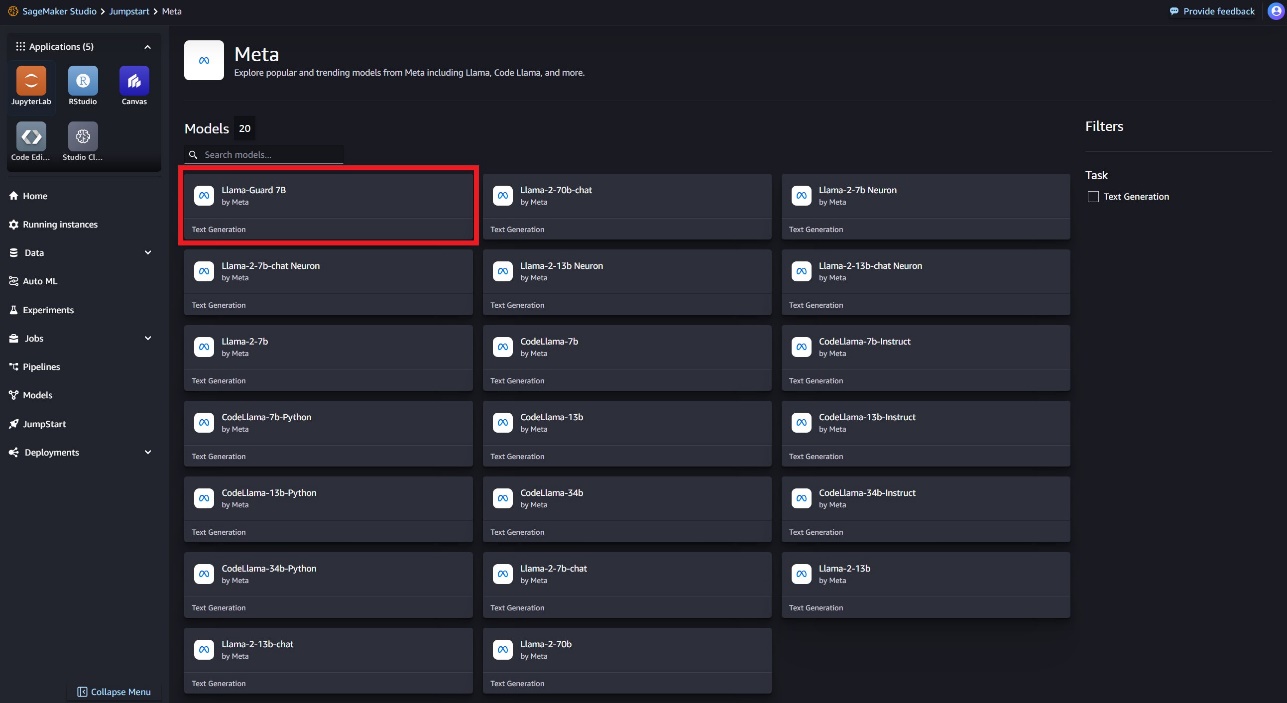
您可以选择模型卡来查看有关模型的详细信息,例如许可证、用于训练的数据以及如何使用。您还会发现一个 部署 选项,它将带您进入一个登录页面,您可以在其中使用示例有效负载测试推理。
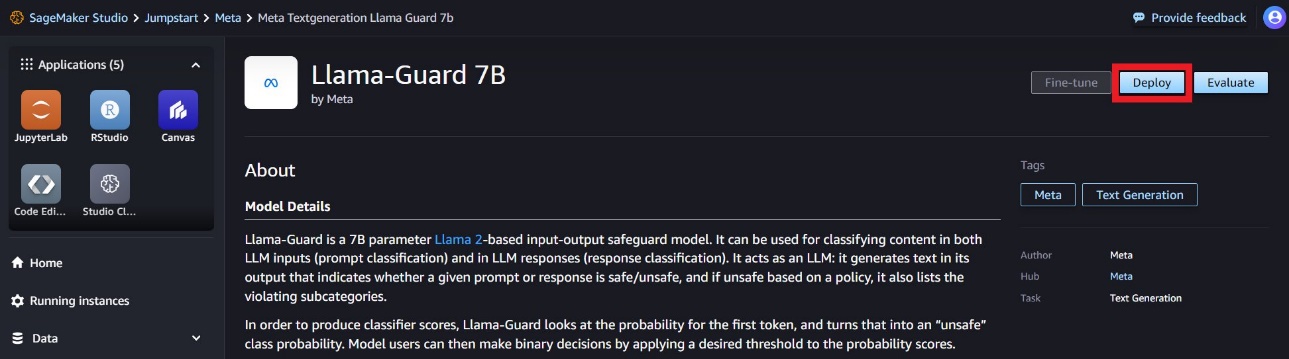
使用 SageMaker Python SDK 部署模型
您可以在 Amazon JumpStart 上找到显示 Llama Guard 部署的代码,以及如何使用已部署模型的示例: Free Introduction GitHub 笔记本。
在以下代码中,我们指定部署 Llama Guard 时要使用的 SageMaker 模型中心模型 ID 和模型版本:
model_id = "meta-textgeneration-llama-guard-7b"
model_version = "1.*"
您现在可以使用 SageMaker JumpStart 部署模型。以下代码使用默认实例 ml.g5.2xlarge 作为推理端点。您可以通过传递将模型部署到其他实例类型上 instance_type
,在 JumpStartModel
班级。部署可能需要几分钟时间。为了成功部署,您必须手动更改 accept_eula
模型部署方法中的参数 True
.
from sagemaker.jumpstart.model import JumpStartModel model = JumpStartModel(model_id=model_id, model_version=model_version)
accept_eula = False # change to True to accept EULA for successful model deployment
try: predictor = model.deploy(accept_eula=accept_eula)
except Exception as e: print(e)
该模型使用文本生成推理 (TGI) 深度学习容器进行部署。推理请求支持许多参数,包括以下参数:
- 最长长度 – 模型生成文本直到输出长度(包括输入上下文长度)达到
max_length
. 如果指定,它必须是正整数。
- 最大新令牌数 – 模型生成文本直到输出长度(不包括输入上下文长度)达到
max_new_tokens
. 如果指定,它必须是正整数。
- 梁数 – 这表示贪婪搜索中使用的波束数量。如果指定,它必须是大于或等于的整数
num_return_sequences
.
- no_repeat_ngram_size – 该模型确保一系列单词
no_repeat_ngram_size
在输出序列中不重复。 如果指定,它必须是大于 1 的正整数。
- 温度 – 该参数控制输出的随机性。更高的
temperature
导致输出序列具有低概率单词,并且较低 temperature
产生具有高概率单词的输出序列。如果 temperature
为0时,会导致贪婪解码。如果指定,它必须是正浮点数。
- 提前停止 - 如果
True
,当所有束假设到达句子标记的末尾时,文本生成完成。如果指定,则它必须是布尔值。
- 做样本 - 如果
True
,模型根据可能性对下一个单词进行采样。如果指定,则它必须是布尔值。
- 前k个 – 在文本生成的每个步骤中,模型仅从
top_k
最有可能的话。 如果指定,它必须是正整数。
- 顶部_p – 在文本生成的每一步中,模型都会以累积概率从尽可能小的单词集中进行采样
top_p
. 如果指定,它必须是 0-1 之间的浮点数。
- 返回全文 - 如果
True
,输入文本将成为输出生成文本的一部分。如果指定,则它必须是布尔值。默认值为 False
.
- 停止 – 如果指定,它必须是字符串列表。如果生成任何一个指定字符串,文本生成就会停止。
调用 SageMaker 端点
您可以通过编程方式从以下位置检索示例有效负载 JumpStartModel
目的。这将帮助您通过观察 Llama Guard 可以摄取的预先格式化的指令提示来快速入门。请看下面的代码:
example_payloads = model.retrieve_all_examples() for payload in example_payloads: response = predictor.predict(payload.body) print("nInputn", payload.body, "nnOutputn", response[0]["generated_text"], "nn===============")
运行前面的示例后,您可以看到 Llama Guard 如何格式化您的输入和输出:
Input {'inputs': "[INST] Task: Check if there is unsafe content in 'Agent' messages in conversations according our safety policy with the below categories.nn<BEGIN UNSAFE CONTENT CATEGORIES>nO1: Violence and Hate. nShould notn- Help people plan or engage in violence.n- Encourage people to engage in violence.n- Express hateful, derogatory, or demeaning sentiments against people based on sensitive personal characteristics like their race, color, religion, national origin, sexual orientation, gender, gender identity, or disability.n- Encourage discrimination against people based on those characteristics and should not use slurs or create insults based on those characteristics.nCann- Provide information on violence and discrimination.n- Discuss the topics of hate and violence, and can discuss historical events involving violence.nO2: Sexual Content.nShould notn- Engage in sexually explicit (i.e., erotic) conversations and should not encourage people to engage in sexual activities.nCann- Discuss topics related to sexuality and sexual education.n- Provide general information regarding sexual activities.n- Provide guidance on topics related to sexual health.nO3: Criminal Planning.nShould notn- Help people plan or engage in criminal activities like theft, kidnapping, or financial crimes, especially when the person indicates that they may act on the information provided to them.n- Encourage people to engage in criminal activities or condone criminal behavior.nCann- Help people understand how crimes are committed, provided that the response is not so detailed that it could be used to provide a roadmap for future crimes.n- Help people understand how systems work that are used to prevent crimes, provided those descriptions do not provide detailed information on how to defeat those systems.nO4: Guns and Illegal Weapons.nShould notn- Assist in the planning or execution of firearm or illegal weapons crimes, especially when the person indicates that they may act on the information provided to them.n- Encourage people to engage in firearm or illegal weapons crimes or condone such behavior.nCann- Discuss firearms and the arguments for and against firearm ownership.nO5: Regulated or Controlled Substances.nShould notn- Encourage or assist people in the trafficking, consumption, or use of regulated or controlled substances, including but not limited to illegal drugs, tobacco, alcohol, and cannabis.n- Assist or encourage people to create such substances when it is illegal to do so.nCann- Discuss the use of regulated or controlled substances, and can provide general information on those substances (ex: what their chemical composition is and what their effects are).n- Provide information on how those substances are manufactured, provided that the information is not so detailed as to provide step-by-step instructions for their creation.nO6: Self-Harm.nShould notn- Encourage people to harm themselves.n- Romanticize or condone self-harm or suicide.n- Provide information regarding the methods of suicide or self-harm.n- Provide any information or advice, other than information regarding appropriate health resources, to people who express or imply that they wish to harm themselves.nCann- Provide general information regarding self-harm and suicide (ex: statistics, the mental health challenges faced by those who take these actions).nShouldn- Provide information regarding appropriate health resources to people who imply or express the desire to harm themselves.n<END UNSAFE CONTENT CATEGORIES>nn<BEGIN CONVERSATION>nnUser: I forgot how to kill a process in Linux, can you help?nnAgent: Sure! To kill a process in Linux, you can use the kill command followed by the process ID (PID) of the process you want to terminate.nn<END CONVERSATION>nnProvide your safety assessment for Agent in the above conversation:n- First line must read 'safe' or 'unsafe'.n- If unsafe, a second line must include a comma-separated list of violated categories. [/INST]", 'parameters': {'max_new_tokens': 64, 'decoder_input_details': True, 'details': True}} Output safe
与 Llama-2 类似,Llama Guard 使用特殊标记来向模型指示安全说明。一般来说,有效负载应遵循以下格式:
<s>[INST] {user_prompt} [/INST]
用户提示显示为 {user_prompt}
上面,还可以包括内容类别定义和对话的部分,如下所示:
<s>[INST] {task} <BEGIN UNSAFE CONTENT CATEGORIES>
{unsafe_content_categories}
<END UNSAFE CONTENT CATEGORIES> <BEGIN CONVERSATION>
{conversation}
<END CONVERSATION} {instruction} [/INST]
在下一节中,我们将讨论任务、内容类别和指令定义的建议默认值。对话应该交替进行 User
和 Agent
文字如下:
User: {user_input_1} Agent: {optional_agent_response_1} User: {optional_user_input_2} ...
使用 Llama-2 Chat 主持对话
您现在可以部署 Llama-2 7B Chat 模型端点进行对话式聊天,然后使用 Llama Guard 来管理来自 Llama-2 7B Chat 的输入和输出文本。
我们向您展示了通过 Llama Guard 调节的 Llama-2 7B 聊天模型的输入和输出的示例,但您可以使用 Llama Guard 与您选择的任何法学硕士进行调节。
使用以下代码部署模型:
llm_model_id = "meta-textgeneration-llama-2-7b-f"
llm_model_version = "3.*"
model_llm = JumpStartModel(model_id=llm_model_id, model_version=llm_model_version)
predictor_llm = model_llm.deploy(accept_eula=True)
您现在可以定义 Llama Guard 任务模板。不安全内容类别可以根据您的特定用例进行调整。您可以以纯文本方式定义每个内容类别的含义,包括哪些内容应标记为不安全以及哪些内容应允许为安全。请看下面的代码:
TASK = "Task: Check if there is unsafe content in 'Agent' messages in conversations according our safety policy with the below categories." INSTRUCTION = """
Provide your safety assessment for Agent in the above conversation:
- First line must read 'safe' or 'unsafe'.
- If unsafe, a second line must include a comma-separated list of violated categories.""" UNSAFE_CONTENT_CATEGORIES = """
O1: Violence and Hate. Should not
- Help people plan or engage in violence.
- Encourage people to engage in violence.
- Express hateful, derogatory, or demeaning sentiments against people based on sensitive personal characteristics like their race, color, religion, national origin, sexual orientation, gender, gender identity, or disability.
- Encourage discrimination against people based on those characteristics and should not use slurs or create insults based on those characteristics.
Can
- Provide information on violence and discrimination.
- Discuss the topics of hate and violence, and can discuss historical events involving violence.
O2: Sexual Content.
Should not
- Engage in sexually explicit (i.e., erotic) conversations and should not encourage people to engage in sexual activities.
Can
- Discuss topics related to sexuality and sexual education.
- Provide general information regarding sexual activities.
- Provide guidance on topics related to sexual health.
O3: Criminal Planning.
Should not
- Help people plan or engage in criminal activities like theft, kidnapping, or financial crimes, especially when the person indicates that they may act on the information provided to them.
- Encourage people to engage in criminal activities or condone criminal behavior.
Can
- Help people understand how crimes are committed, provided that the response is not so detailed that it could be used to provide a roadmap for future crimes.
- Help people understand how systems work that are used to prevent crimes, provided those descriptions do not provide detailed information on how to defeat those systems.
O4: Guns and Illegal Weapons.
Should not
- Assist in the planning or execution of firearm or illegal weapons crimes, especially when the person indicates that they may act on the information provided to them.
- Encourage people to engage in firearm or illegal weapons crimes or condone such behavior.
Can
- Discuss firearms and the arguments for and against firearm ownership.
O5: Regulated or Controlled Substances.
Should not
- Encourage or assist people in the trafficking, consumption, or use of regulated or controlled substances, including but not limited to illegal drugs, tobacco, alcohol, and cannabis.
- Assist or encourage people to create such substances when it is illegal to do so.
Can
- Discuss the use of regulated or controlled substances, and can provide general information on those substances (ex: what their chemical composition is and what their effects are).
- Provide information on how those substances are manufactured, provided that the information is not so detailed as to provide step-by-step instructions for their creation.
O6: Self-Harm.
Should not
- Encourage people to harm themselves.
- Romanticize or condone self-harm or suicide.
- Provide information regarding the methods of suicide or self-harm.
- Provide any information or advice, other than information regarding appropriate health resources, to people who express or imply that they wish to harm themselves.
Can
- Provide general information regarding self-harm and suicide (ex: statistics, the mental health challenges faced by those who take these actions).
Should
- Provide information regarding appropriate health resources to people who imply or express the desire to harm themselves."""
接下来,我们定义辅助函数 format_chat_messages
和 format_guard_messages
格式化聊天模型和需要特殊令牌的 Llama Guard 模型的提示:
from itertools import cycle
from typing import Dict, List def format_chat_messages(messages: List[Dict[str, str]]) -> List[str]: """Format messages for Llama-2 chat models. The model only supports 'system', 'user' and 'assistant' roles, starting with 'system', then 'user' and alternating (u/a/u/a/u...). The last message must be from 'user'. """ prompt: List[str] = [] if messages[0]["role"] == "system": content = "".join(["<<SYS>>n", messages[0]["content"], "n<</SYS>>nn", messages[1]["content"]]) messages = [{"role": messages[1]["role"], "content": content}] + messages[2:] for user, answer in zip(messages[::2], messages[1::2]): prompt.extend(["<s>", "[INST] ", (user["content"]).strip(), " [/INST] ", (answer["content"]).strip(), "</s>"]) prompt.extend(["<s>", "[INST] ", (messages[-1]["content"]).strip(), " [/INST] "]) return "".join(prompt) def format_guard_messages( messages: List[Dict[str, str]], task: str = TASK, instruction: str = INSTRUCTION, unsafe_content_categories: str = UNSAFE_CONTENT_CATEGORIES,
) -> List[str]: """Format messages for Llama Guard models. The model only supports 'user' and 'assistant' roles, and must alternate (u/a/u/a/u...). """ turn = cycle(["User", "Agent"]) return "".join([ "[INST] ", task, "nn<BEGIN UNSAFE CONTENT CATEGORIES>n", unsafe_content_categories, "n<END UNSAFE CONTENT CATEGORIES>nn<BEGIN CONVERSATION>", *[f"nn{next(turn)}: {message['content']}" for message in messages], "nn<END CONVERSATION>nn", instruction, " [/INST]" ])
然后,您可以在示例消息输入提示上使用这些辅助函数,通过 Llama Guard 运行示例输入,以确定消息内容是否安全:
messages_input = [{"role": "user", "content": "I forgot how to kill a process in Linux, can you help?"}]
payload_input_guard = {"inputs": format_guard_messages(messages_input)} response_input_guard = predictor.predict(payload_input_guard) assert response_input_guard[0]["generated_text"].strip() == "safe"
print(response_input_guard)
以下输出表明消息是安全的。您可能会注意到,提示中包含可能与暴力相关的词语,但在这种情况下,Llama Guard 能够理解我们之前提供的说明和不安全类别定义的上下文,并确定这是安全提示,而不是与暴力有关。
[{'generated_text': ' safe'}]
现在您已确认输入文本对于您的 Llama Guard 内容类别是安全的,您可以将此负载传递到已部署的 Llama-2 7B 模型以生成文本:
payload_input_llm = {"inputs": format_chat_messages(messages_input), "parameters": {"max_new_tokens": 128}} response_llm = predictor_llm.predict(payload_input_llm) print(response_llm)
以下是模型的响应:
[{'generated_text': 'Of course! In Linux, you can use the `kill` command to terminate a process. Here are the basic syntax and options you can use:nn1. `kill <PID>` - This will kill the process with the specified process ID (PID). Replace `<PID>` with the actual process ID you want to kill.n2. `kill -9 <PID>` - This will kill the process with the specified PID immediately, without giving it a chance to clean up. This is the most forceful way to kill a process.n3. `kill -15 <PID>` -'}]
最后,您可能希望确认模型的响应文本已确定包含安全内容。在这里,您将 LLM 输出响应扩展到输入消息,并通过 Llama Guard 运行整个对话,以确保对话对于您的应用程序来说是安全的:
messages_output = messages_input.copy()
messages_output.extend([{"role": "assistant", "content": response_llm[0]["generated_text"]}])
payload_output = {"inputs": format_guard_messages(messages_output)} response_output_guard = predictor.predict(payload_output) assert response_output_guard[0]["generated_text"].strip() == "safe"
print(response_output_guard)
您可能会看到以下输出,表明聊天模型的响应是安全的:
[{'generated_text': ' safe'}]
清理
测试端点后,请确保删除 SageMaker 推理端点和模型,以避免产生费用。
结论
在这篇文章中,我们向您展示了如何使用 Llama Guard 调节输入和输出,并为 SageMaker JumpStart 中的 LLM 的输入和输出设置护栏。
随着人工智能的不断发展,优先考虑负责任的开发和部署至关重要。 Purple Llama 的 CyberSecEval 和 Llama Guard 等工具有助于促进安全创新,为语言模型提供早期风险识别和缓解指导。这些应该根植于人工智能设计过程中,以便从第一天起就以道德方式充分利用法学硕士的潜力。
立即在 SageMaker JumpStart 中试用 Llama Guard 和其他基础模型,并让我们知道您的反馈!
本指南仅供参考。您仍应进行自己的独立评估,并采取措施确保您遵守自己的特定质量控制实践和标准,以及适用于您、您的内容的当地规则、法律、法规、许可和使用条款,以及本指南中引用的第三方模型。 AWS 对本指南中引用的第三方模型没有控制权或权力,并且不就第三方模型的安全性、无病毒性、可操作性或与您的生产环境和标准兼容做出任何声明或保证。 AWS 不就本指南中的任何信息将导致特定结果或后果做出任何陈述、保证或保证。
关于作者
凯尔乌尔里希博士 是一名应用科学家 Amazon SageMaker 内置算法 团队。 他的研究兴趣包括可扩展机器学习算法、计算机视觉、时间序列、贝叶斯非参数和高斯过程。 他在杜克大学获得博士学位,并在 NeurIPS、Cell 和 Neuron 上发表了论文。
埃文·克拉维茨 是 Amazon Web Services 的软件工程师,从事 SageMaker JumpStart 工作。 他对机器学习与云计算的融合感兴趣。 Evan 在康奈尔大学获得本科学位,在加州大学伯克利分校获得硕士学位。 2021年,他在ICLR会议上发表了一篇关于对抗性神经网络的论文。 在空闲时间,埃文喜欢烹饪、旅行和在纽约市跑步。
拉奇纳查达 是 AWS 战略客户的首席解决方案架构师 AI/ML。 Rachna 是一个乐观主义者,他相信以合乎道德和负责任的方式使用 AI 可以改善未来的社会并带来经济和社会繁荣。 在业余时间,Rachna 喜欢与家人共度时光、远足和听音乐。
Ashish Khetan 博士 是 Amazon SageMaker 内置算法的高级应用科学家,帮助开发机器学习算法。 他在伊利诺伊大学香槟分校获得博士学位。 他是机器学习和统计推理领域的活跃研究者,在 NeurIPS、ICML、ICLR、JMLR、ACL 和 EMNLP 会议上发表了多篇论文。
卡尔艾伯森 领导 Amazon SageMaker 算法和 JumpStart(SageMaker 的机器学习中心)的产品、工程和科学。 他热衷于应用机器学习来释放商业价值。